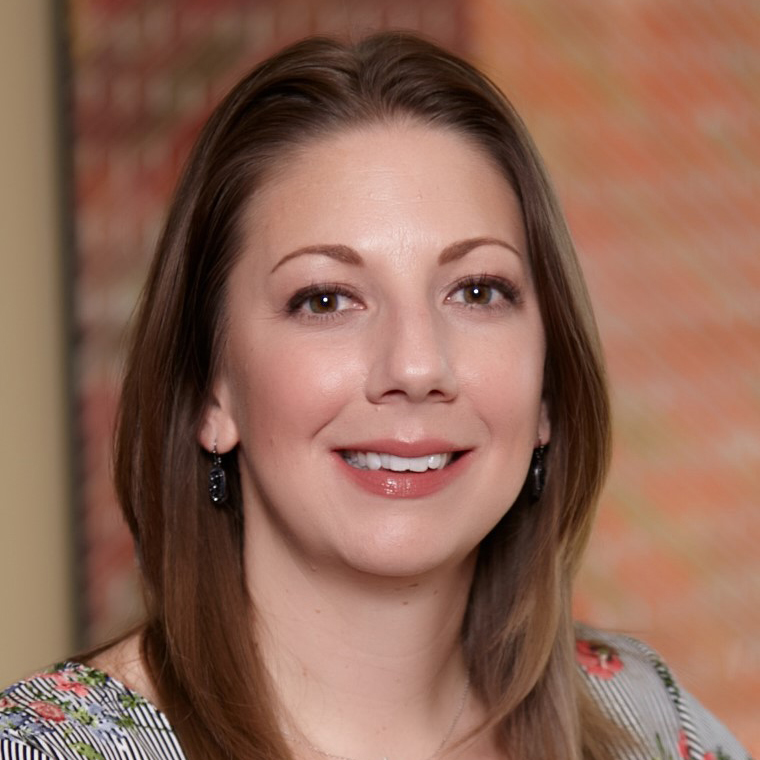
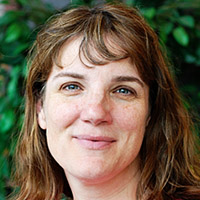
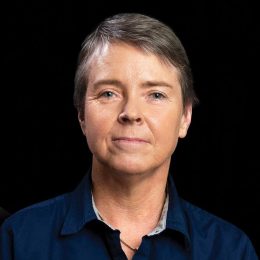
Kristin Flaming (Valdosta State University), Lisa Dierker (Wesleyan University), Jennifer Rose (Wesleyan University)
Abstract
Background. A central challenge of introductory statistics courses is the development of a curriculum that not only serves diverse students but also sparks communication, reasoning, and collaboration that crosses traditional disciplinary boundaries. The “tools” that students are commonly provided often do not serve them well given real-world challenges or convey the same “rich, complicated context, and decision-making issues present in the experience of real application” (Nolan & Temple Lang, 2009). There is an emerging literature base that demonstrates that project-based learning is more effective in promoting deep thinking—the ability to apply knowledge, communication, and reasoning skills—when compared to traditional didactic approaches (e.g., Hmelo-Silver et al., 2007; Walker & Leary, 2009). Passion-Driven Statistics (https://passiondrivenstatistics.com) is a project-based, introductory statistics curriculum that supports students in conducting original research with real-world data. Funded by NSF, the curriculum follows the recommendations outlined in the Guidelines for Assessment and Instruction in Statistics Education report (Carver et al., 2016). Students pose exciting research questions that matter to them, then discover how to answer those questions using real data and statistics. In pursuing these answers, students also learn basic statistical programming in a software platform of their instructors' choosing (e.g., SAS, R, SPSS, Stata, Python). Methods. Researchers compared background characteristics, learning experiences and course outcomes for students completing the project-based course compared to those completing a traditional introductory statistics course. Both were semester-long courses meeting 2 to 3 times a week and were open to all students with no prerequisites for enrollment. Data were drawn from administrative records and student surveys completed before and after each course. Chi-Square Tests of Independence with odds ratios and ANOVA with Cohen’s d were calculated to examine the associations. Multivariate regression models were then run to evaluate whether differences between courses persisted when controlling for student background characteristics. Findings. Students enrolled in the course had more positive course experiences than those enrolled in a traditional course including better understanding the information presented through one-on-one support, engaging in greater preparation for class, finding the course more useful and gauging its reward and feelings of accomplishment more highly (Dierker et al., 2018). It has been found to be associated with increases in confidence and interest in pursuing additional coursework in data analysis and statistics (Dierker et al., 2018). Similar findings with the curriculum on undergraduate students in Ghana, West Africa, demonstrate the potential for its global portability (Awuah et al., 2020). Implications. By making data science education more accessible, the project aims to increase the recruitment and retention of women and other underrepresented students into careers requiring data analysis skills. In this way, it can help to create a larger, more diverse population with the data analysis skills needed across industry sectors, disciplines, and audiences, thus contributing to the nation's competitiveness in the global economy. By conducting evaluative research that includes the areas of educator training, program sustainability, and student outcomes, the project will contribute new knowledge about teaching and learning data analytics.