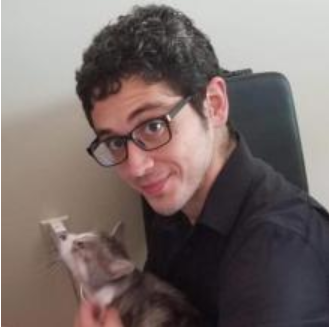
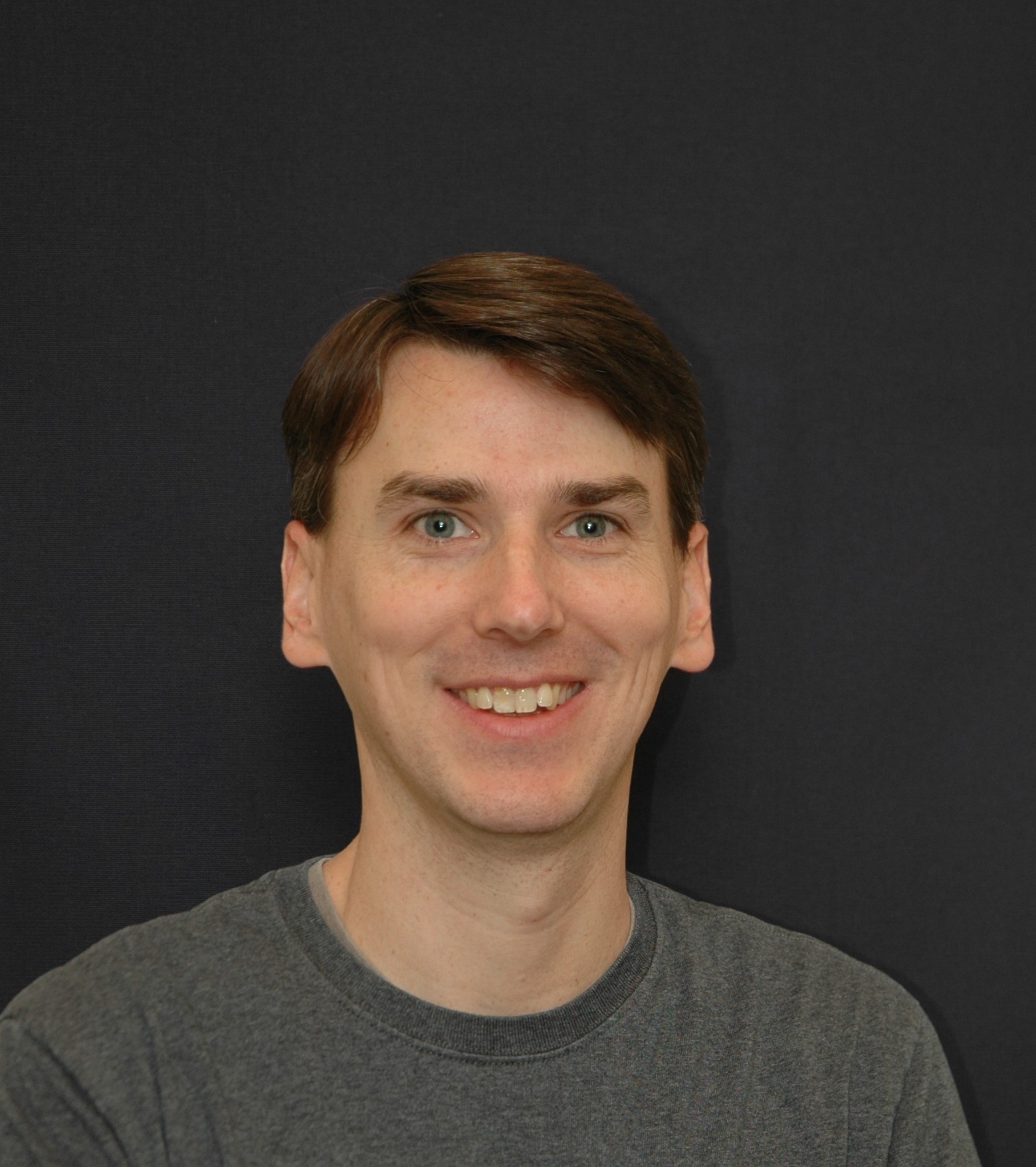
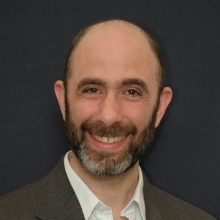
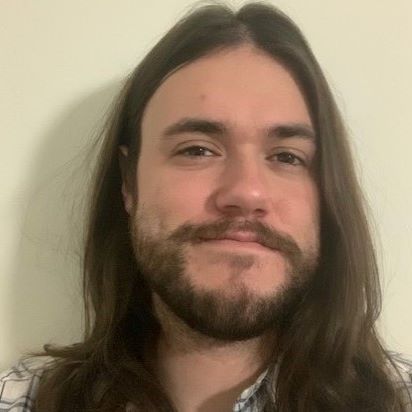
John Vargas (University of Massachusetts Amherst),Jeffrey Starns (UMass Amherst), Andrew Cohen (UMass Amherst), Michael Tuttle (UMass Amherst)
Abstract
Bayesian inference – a procedure for updating the probability that a hypothesis is true in response to new data – is quickly gaining popularity as a statistical and modeling methodology but is frequently excluded from statistics courses. Given the already taxing demands of statistical education, instructors may feel hesitant to introduce the additional complexity of Bayesian concepts. The goals of this workshop are to (1) describe a strategy for integrating Bayesian concepts into existing statistics courses with minimal class restructuring, (2) introduce instructional methods supported by a novel interactive spatial technique for performing Bayesian inference that explicitly represents the underlying logic of Bayesian updating, and (3) present data on ways that the spatial technique can enhance learning of statistical concepts. No prior knowledge is required for participation, and the issues we discuss will be relevant to faculty and students who want to learn more about Bayesian methods as well as instructors who are well-versed in Bayesian methods but uncertain of how to best present them to students. Attendees will be asked to use their personal computers to access the education materials that we will share. Included in those materials is a desktop application that introduces our novel spatial visualization in an interactive medium. This application requires only a web browser. Attendees may also want to engage with programming scripts (using R studio) at their own discretion.