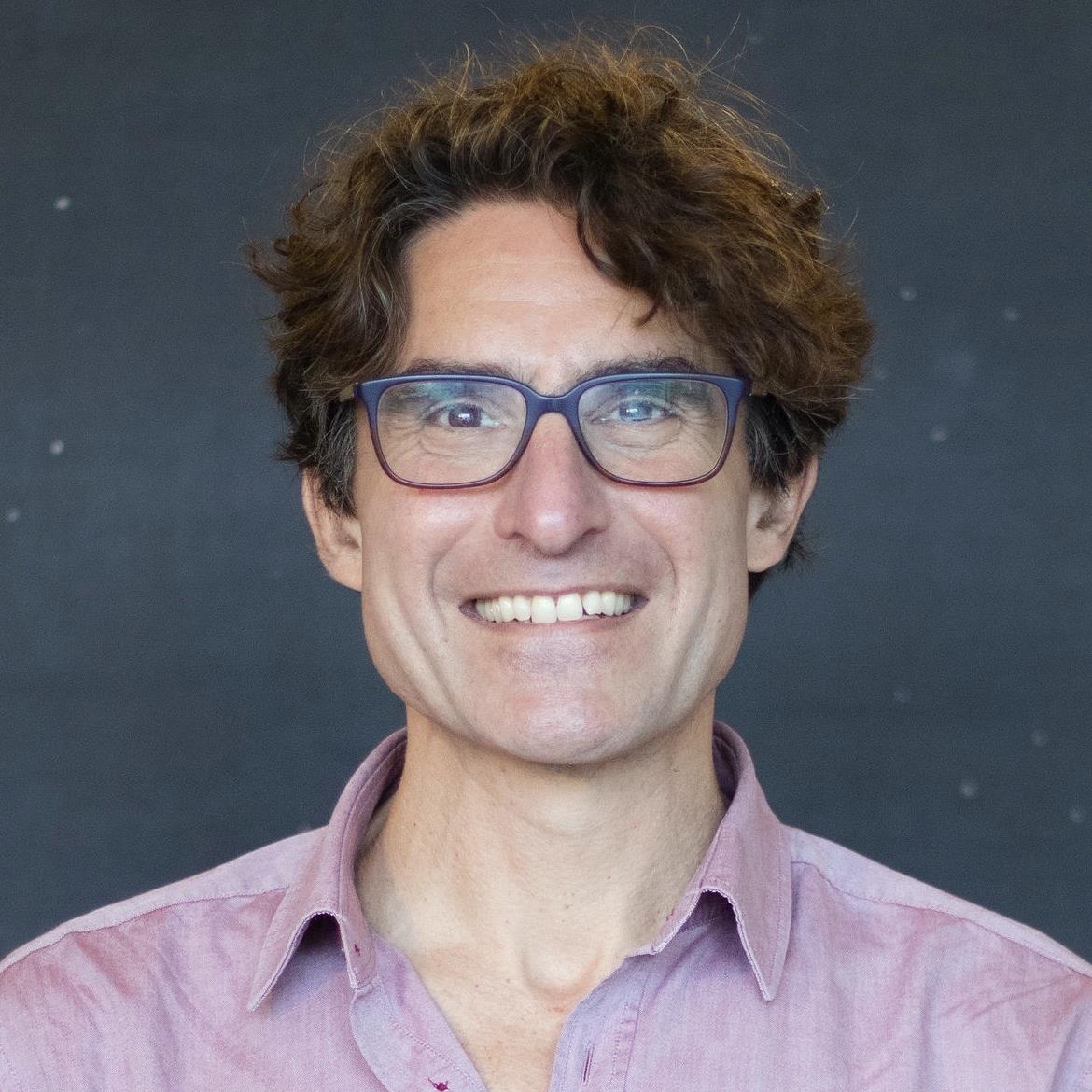
Nathan Taback (University of Toronto)
Abstract
Tukey and Wilk (1966) described characteristics shared by data analysis and experimentation as “… an open-ended, highly interactive, iterative process, whose actual steps are selected segments of a stubbily branching, tree-like pattern of possible actions.” A key skill for modern data scientists and statisticians is the ability to experiment on raw data. How can we teach data analysis as experimentation? Some possibilities include teaching students to explore fitting different models to the same data set, and another is to explore fitting a model to different data sets that arise from alternatively processed data sets based on feasible options for variable transformation, and data exclusion. The latter provides a framework for teaching statistics as an interactive, iterative process of problem-solving via data-wrangling—another important skill for students. Students gain experience developing feasible choices for converting raw data into analysis data which in turn gives rise to a multiverse of statistical results (Steegen et al. 2016), allowing students to examine the robustness of a finding. In this talk I will introduce multiverse analysis as a framework for teaching experimentation on raw data, describe how instructors might incorporate multiverse analysis into statistics or data science courses using mverse¬—a new R package developed for teaching multiverse analysis.