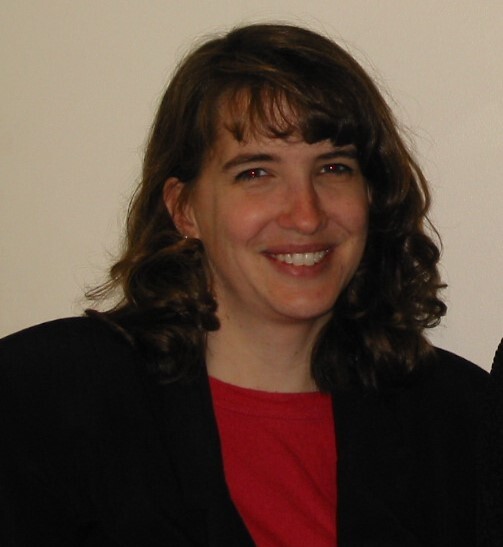
Friday Morning, July 18th
- Speaker: Beth Chance (Cal Poly - San Luis Obispo)
- Title: Teaching about models: A unifying topic?
- Abstract: Oftentimes, the introductory statistics course can feel like a collection of unrelated topics to novice students. Joe Ward and others have long advocated centering the introductory statistics course around modeling. With improvements in the accessibility and effectiveness of new technology tools for younger students, recent materials in introductory statistics have encouraged a refocus on statistical modeling (e.g., Kaplan, Gelman, CourseKata, Catalysts for Change). I agree with this need, but confess I have not yet fully adopted these approaches. However, rather than thinking of instruction on modeling as introducing a new topic or reframing the entire course, perhaps starting with statistical models can provide an opportunity to show students a more unified framework of statistical thinking. In this talk, I will illustrate some small steps that can be taken that don’t require extensive course revisions, new terminology, or mathematical formulas, but focus on helping students first understand the idea of a model. These ideas can be integrated through several topics in the introductory course and then leveraged in later topics and follow-up courses. I will also discuss comparisons we are making in helping students visualize and critique models, and whether such small steps appear to improve students' understanding of larger statistical ideas. Particular focus will be given to data-generating processes, using models to understand data, and simulation models, as well as what we can learn from other disciplines.
- Bio Sketch: Beth Chance is Professor of Statistics at Cal Poly-San Luis Obispo. She is co-author of the Workshop Statistics series, Investigating Statistical Concepts, Applications, and Methods, Introduction to Statistical Investigations and Intermediate Statistical Investigations, all of which adopt an active learning approach to introductory statistics. She has received NSF grants related to innovations in statistics education, helped develop the Rossman/Chance applet collection, and has been involved with the AP Statistics program for many years. She is a Fellow of the American Statistical Association, and recipient of the ASA’s Waller Education Awards, and the Mu Sigma Rho Statistical Education Award.
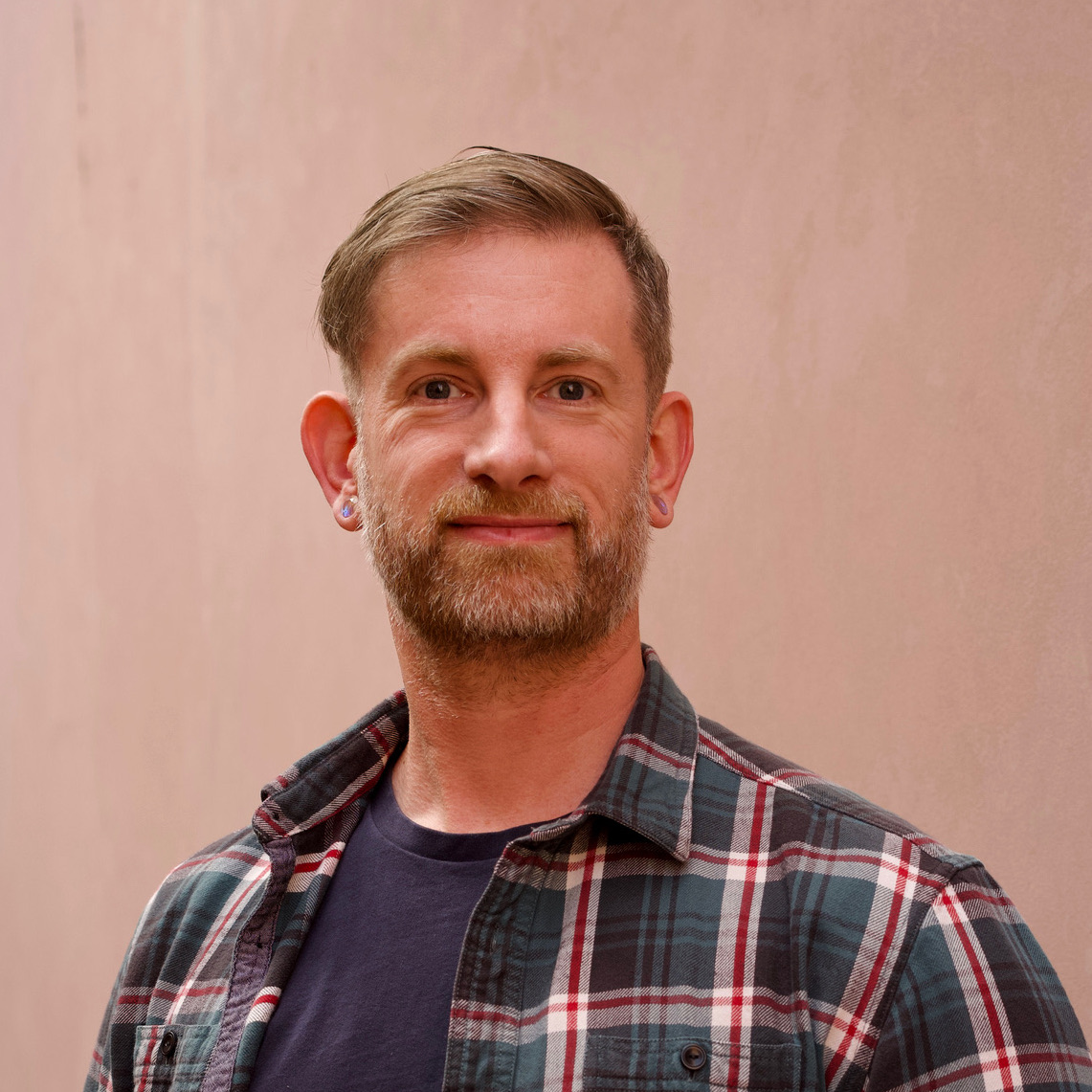
Friday Afternoon, July 18th
- Speaker: Hadley Wickham (Posit)
- Title: Teaching (and learning) the tidyverse with LLMs
- Abstract: TBA
- Bio Sketch: Hadley is Chief Scientist at Posit PBC, winner of the 2019 COPSS award, and a member of the R Foundation. He builds tools (both computational and cognitive) to make data science easier, faster, and more fun. His work includes packages for data science (like the tidyverse, which includes ggplot2, dplyr, and tidyr)and principled software development (e.g. roxygen2, testthat, and pkgdown). He is also a writer, educator, and speaker promoting the use of R for data science. Learn more on his website, http://hadley.nz.
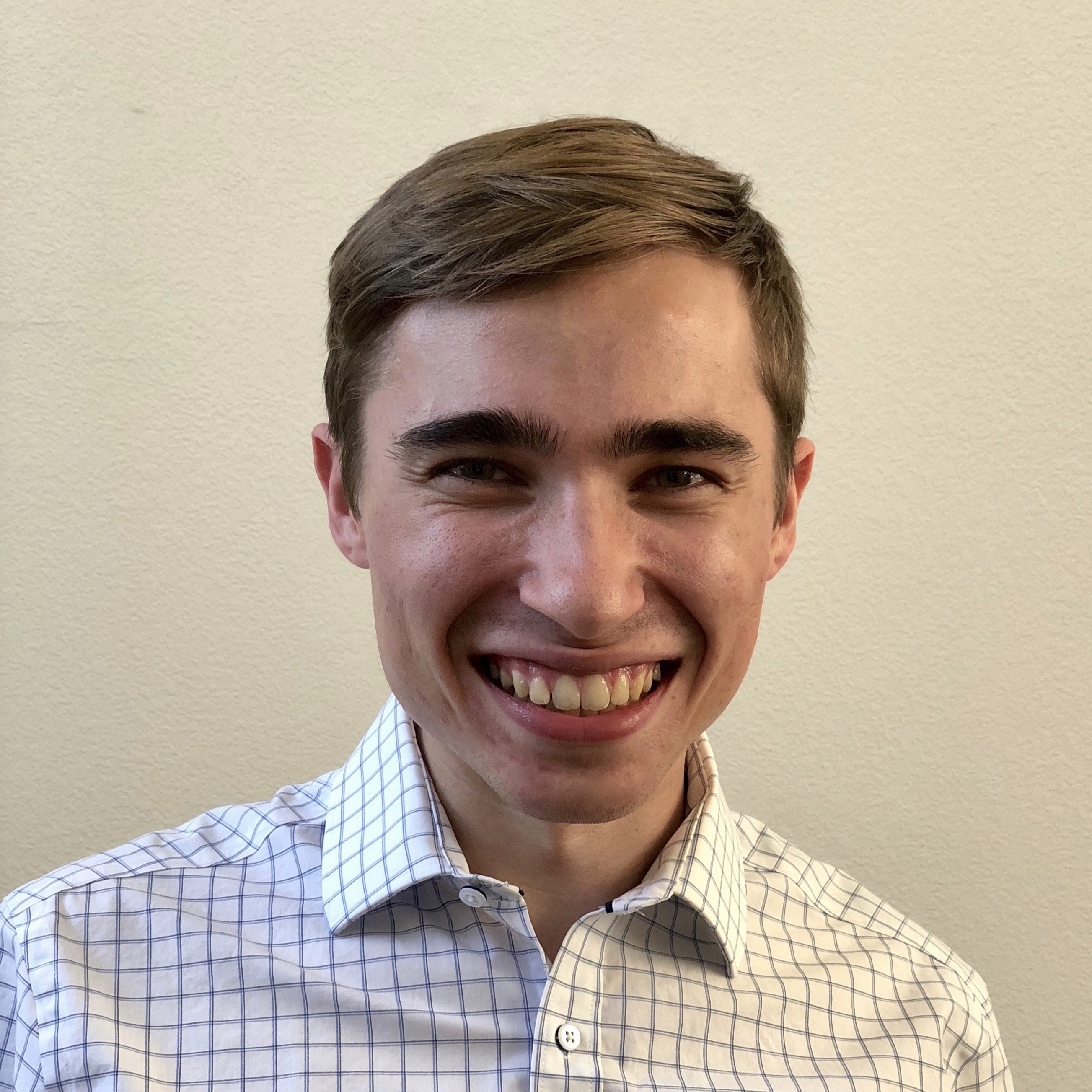
Friday Banquet, July 18th
- Speaker: Zarek Drozda (Data Science 4 Everyone | The University of Chicago)
- Title: Charting the course: Data science in K-12?
- Abstract: What's data got to do with it? The momentum for K-12 Data Science education has increased substantially in recent years: from pilots in school districts, to new software development projects, to changing statewide expectations for students, the landscape is changing quickly. The demand for AI talent and better digital navigation are only increasing the importance of this work. Come hear the case for Data Science (and Data Literacy!) in K-12 education. This session will include the "why," the "what," and the current "where" of K-12 Data Science. Expect some fun, some useful information, and even some controversy as we debate whether the "Data Science" model is the useful one, together.
- Bio Sketch: Zarek Drozda is the Executive Director of Data Science 4 Everyone, a national initiative and coalition based at the University of Chicago. Zarek helped launch DS4E in 2019 with Freakonomics' Steven Levitt, co-organizing a coalition of 3000+ education leaders to advance data science and data literacy education in K-12 schools across more than 25 states. Zarek also contributes to AI education policy with the Federation of American Scientists, and previously served for the U.S. Department of Education.
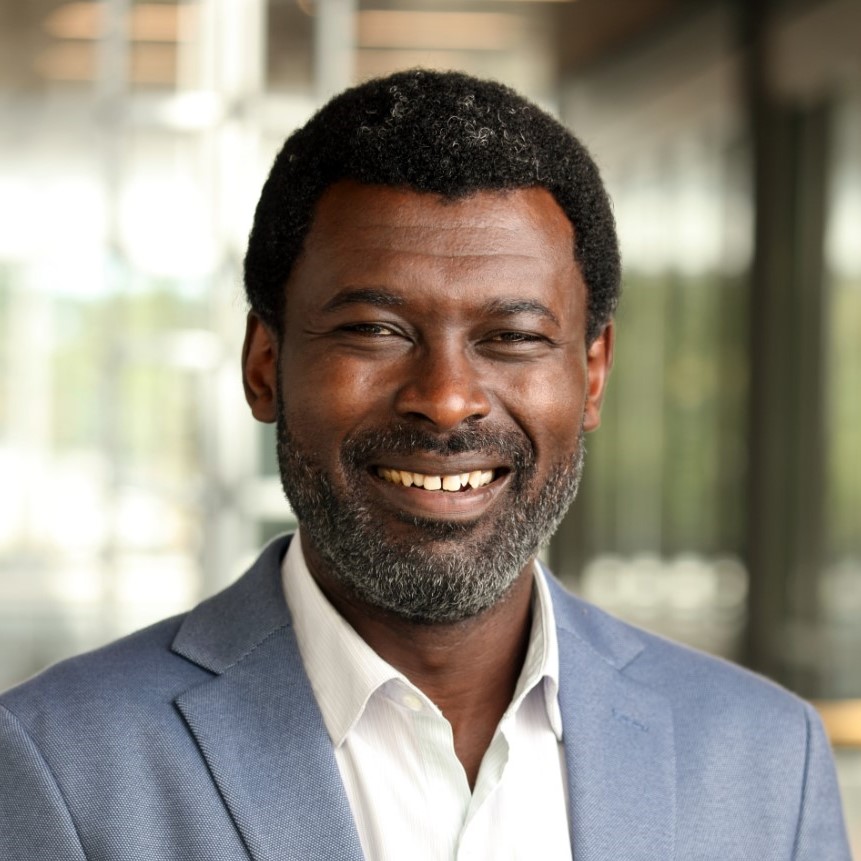
Saturday Morning, July 19th
- Speaker: Prince Afriyie (University of Virginia)
- Title: Students’ “attention is all you need” to transform complexity to clarity
- Abstract: “Attention Is All You Need” is a landmark paper in machine learning authored by scientists at Google in 2017. The ground-breaking paper explains how to train a model using attention as a mechanism that allows it to focus on specific parts of their input. In this session, we will rework this idea in the context of how to direct our students’ attention to help them gain a deeper understanding of useful models. More specifically, we will examine case studies of an essential toolkit of useful models to demonstrate how to absorb our student’s attention through intuition, motivation and model communication. Ultimately, we aim to highlight key attributes that make these models particularly useful in terms of driving value, insights, and decision-making in various application areas.
- Bio Sketch: Prince Afriyie is an associate professor of data science at the University of Virginia’s School of Data Science where he is also the program director of the residential master’s in data science program. Dr. Afriyie’s research includes multiple comparisons as well as statistics and data science education. He currently serves on the Statistics Review Committee for the Centers for Disease Control and Prevention journal, Preventing Chronic Diseases, where he helps to advance understanding and dissemination of statistical methods in the public health field.
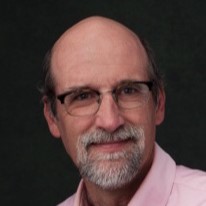
Saturday Afternoon, July 19th
- Speaker: Jeff Witmer (Oberlin College)
- Title: Be effective; don’t be significant
- Abstract: As educators we should be models of good statistical practice for our students. But what is good practice? What should we be teaching to make our courses more useful? How can we help the discipline of statistics continue to evolve? I have a few opinions to share, among them: (1) teach students about the importance of the size of an effect (and not just the size of a p-value); (2) if the p-value is small, then say that we have a discernible effect, rather than calling the result “significant;” and (3) add logistic regression to introductory statistics.
- Bio Sketch: Jeff Witmer is Professor of Statistics at Oberlin College. He is a former Editor of the Journal of Statistics and Data Science Education and operates the Isolated Statisticians listserv. He has co-authored many books, including Stat2: Modeling with Regression and ANOVA, Statistics for the Life Science, and Activity-Based Statistics.