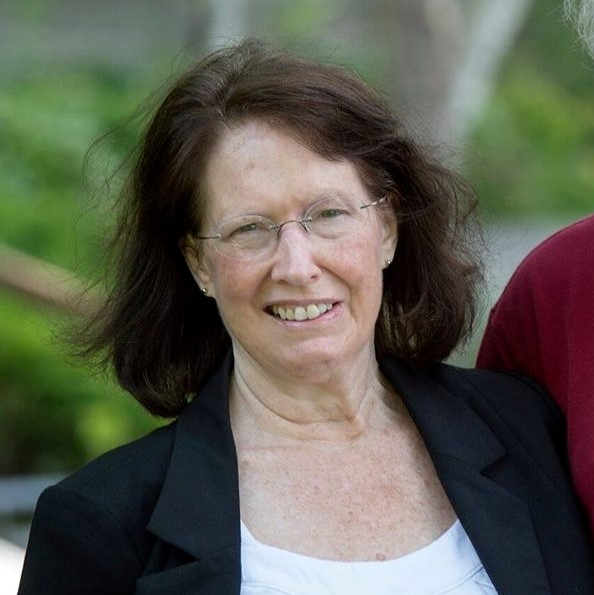
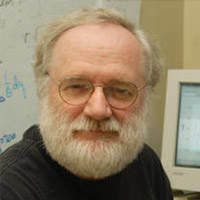
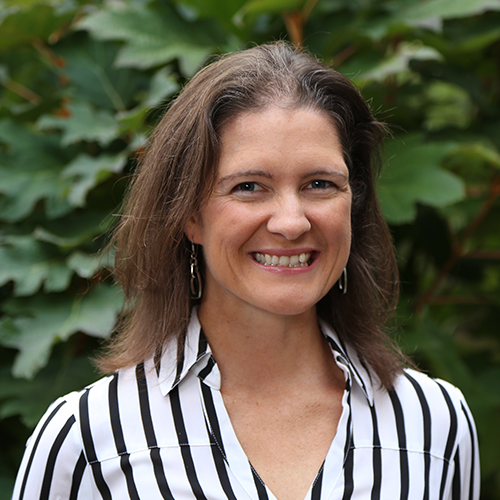
Patti Frazer Lock, Robin Lock (St. Lawrence University); and Kari Lock Morgan (Penn State University)
Schedule
Thursday, May 18 at 1:00 p.m. to Thursday, May 18 at 4:00 p.m.
Abstract
In many traditional approaches to teaching statistical inference, the only connection between the data and an inference procedure is through summary statistics in a computational formula that relies on some approximating distribution. These methods typically require a substantial amount of underlying background material and each parameter situation (mean, proportion, difference, correlation, etc.) needs its own specialized set of derivations. On the other hand, simulation-based inference methods are completely data-driven, relying only on the collected data to generate the distributions needed to do inference. These methods are quite intuitive, offer good visual connections to the key ideas, and can be easily adapted to different situations. They are reflected in the Common Core State Standards for mathematics, but many teachers have little background in some of the concepts, such as “develop a margin of error through the use of simulation models” or “use simulations to decide if differences between parameters are significant.” This is not surprising since these approaches are still fairly novel in undergraduate statistics courses. This workshop will discuss what these methods are, how they work, and how they can be easily incorporated into introductory classes. This session will be especially useful for current high school teachers, those involved with training future mathematics teachers, and those interested in offering intro stat courses that include some simulation-based inference.