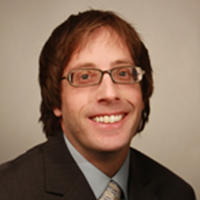
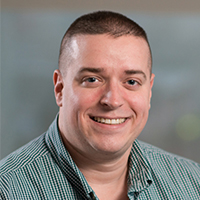
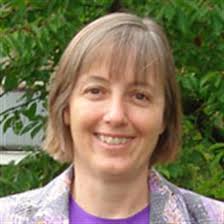
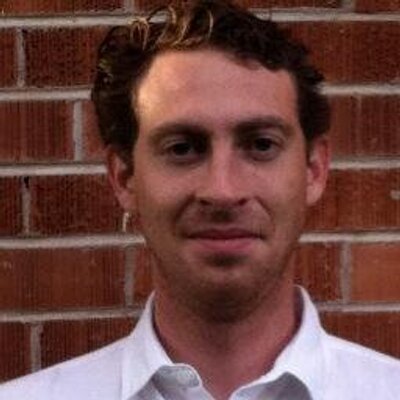
Andrew Zeifler (University Minnesota); Adam Sullivan (Brown University); Jessica Utts (University of California); and Ben Wender (Arizona State University, Tempe)
Abstract
The need to manage, analyze, and extract knowledge from large, complex data sets is growing across industry, government, and academia. Addressing this need requires proper training and education for the workforce; however, it remains unclear whether enough students are being trained to be sufficiently fluent and ready to contribute to a world awash with data. The emerging field of data science may address this critical need. Data science is generally understood as a hybrid of several disciplines, including statistics, computer science, informatics, and mathematics. There are a growing number of data science programs and stand-alone courses. Nonetheless, there is little consensus regarding the essential elements of statistics that should be incorporated into an undergraduate data science course and little discussion of how teaching of these concepts may need to change in the data science context. This session will provide participants with an update on ongoing and recent data science education activities organized by the Committee on Applied and Theoretical Statistics (CATS) of the National Academies of Sciences, Engineering, and Medicine. In this session, participants will take part in a group discussion of key statistical concepts for data science students, and then break in to small working groups to develop a semester-long syllabus for a data science course at either the freshman or advanced undergraduate level. Groups will then report back and discuss commonalities, differences, and themes across the various syllabi developed.