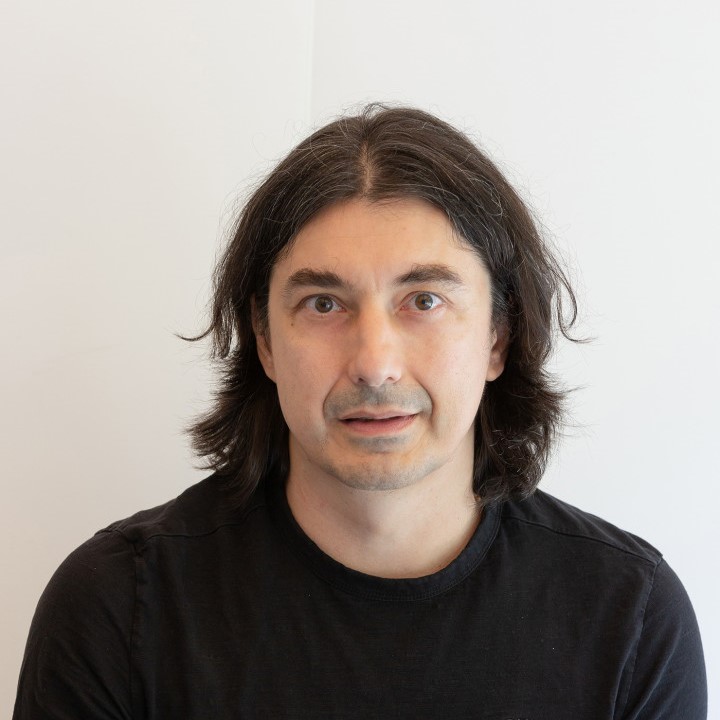
Dobrin Marchev (Columbia University), Banu Baydil (Columbia University)
Abstract
We will share ideas on how to teach advanced statistical concepts and models in the context of an undergraduate Applied Bayesian Statistics course. The considered topics include hierarchical multilevel data structures, missing data and social networks. The talk is based upon authors’ personal experience in creating and teaching a non-Calculus-based Applied Bayesian Statistics course aimed at students who apply Statistics in fields such as social sciences, economics, neuroscience, biology, behavioral science, political science, and similar, and are pursuing a minor in Statistics. Undergraduate students in these disciplines typically learn these advanced topics and concepts in separate courses utilizing complicated methods and techniques for estimation, often finding them perplexing and intimidating in their roles as applied statisticians. As a result, some students are reluctant in utilizing these powerful statistical models or avoid utilizing them at all. We propose a unified methodology on how to handle the complexities of such advanced models by integrating them in one cohesive approach under the umbrella of Bayesian models. The technical side of estimation and inference is implemented in RStan and is demonstrated on real-data examples drawn from fields of study relevant to the students. We aim this talk at undergraduate college instructors who are considering teaching applied Bayesian models with only a minimal assumed knowledge of applied regression models without calculus. On the technical side, R programming skills are useful and bringing a laptop to the session is encouraged. We will have several interactive hands-on activities with opportunities to try the techniques and ask questions.