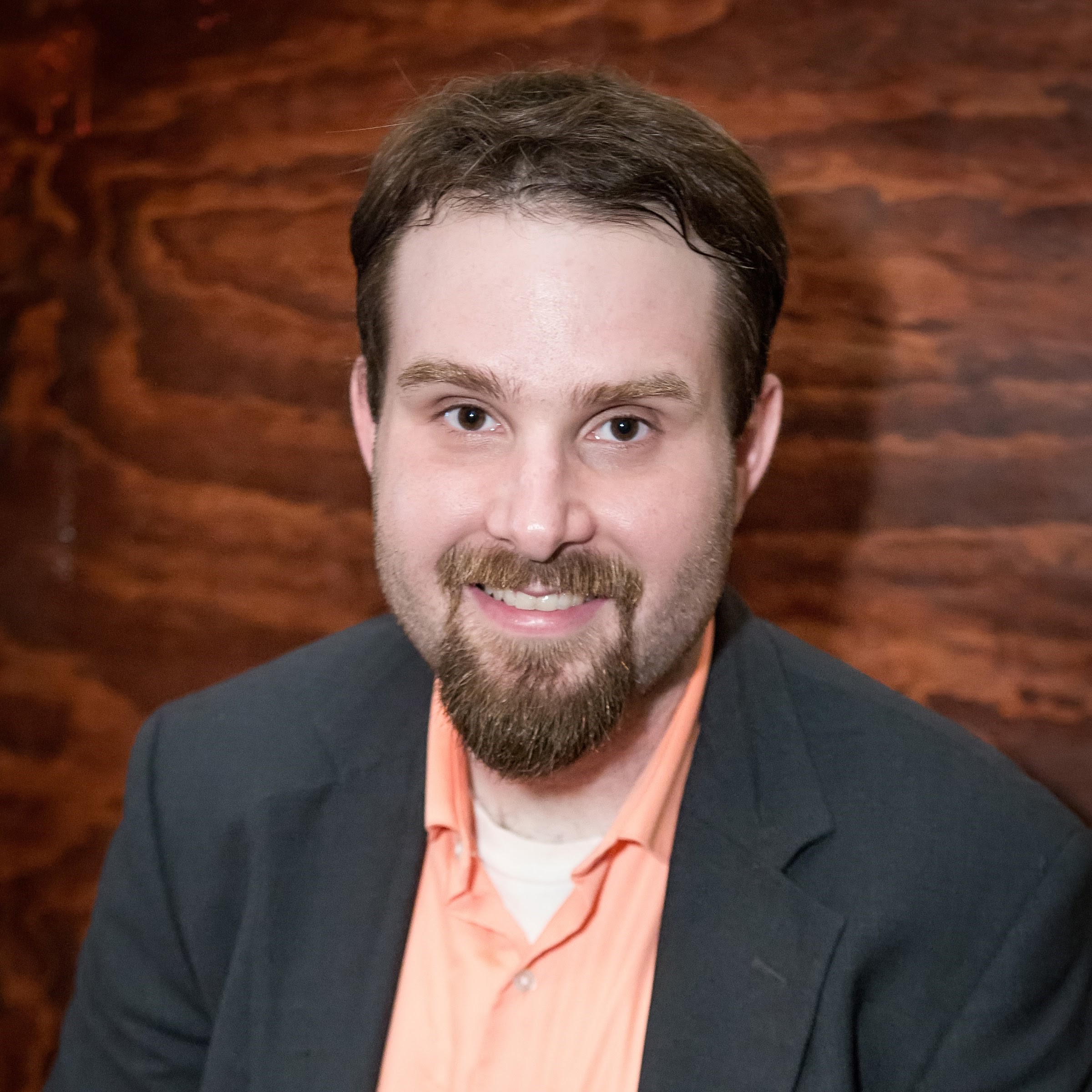
Zachary Binney (Oxford College of Emory University)
Abstract
Many introductory statistics courses currently teach the “grab bag” of standard statistical tests (e.g. independent t-test, paired t-test, ANOVA, and so on). They only reach linear regression at the end. This often leaves students thinking statistics is not a unified framework, but simply a matter of plugging your data into the correct test or model. It may also instill other bad habits such as categorizing continuous data to fit one of these tests. This session proposes a different framework beginning with and centered around linear regression. Each test is equivalent to a linear regression model; for example, ANOVA is simply a linear regression with one categorical predictor using dummy variables (more examples here: https://lindeloev.github.io/tests-as-linear/). We discuss how to introduce linear regression to students with zero statistics background. Then we will work through and discuss an interactive R lesson from the Sports Content for Outreach, Research, and Education (SCORE) network that illustrates how to teach ANOVA using a regression-forward approach with tennis data. By the end of the session participants will hopefully have an understanding of the regression-forward pedagogical model, its pros and cons, ideas for how to deploy it in their own institution’s courses, and an example lesson for ANOVA. The intended audience is anyone teaching any introductory statistics course, though it may be most applicable for those teaching courses that cover the “grab bag” of statistical tests. There is no prerequisite knowledge beyond the content of an introductory statistics course. A laptop running R is suggested but not required.