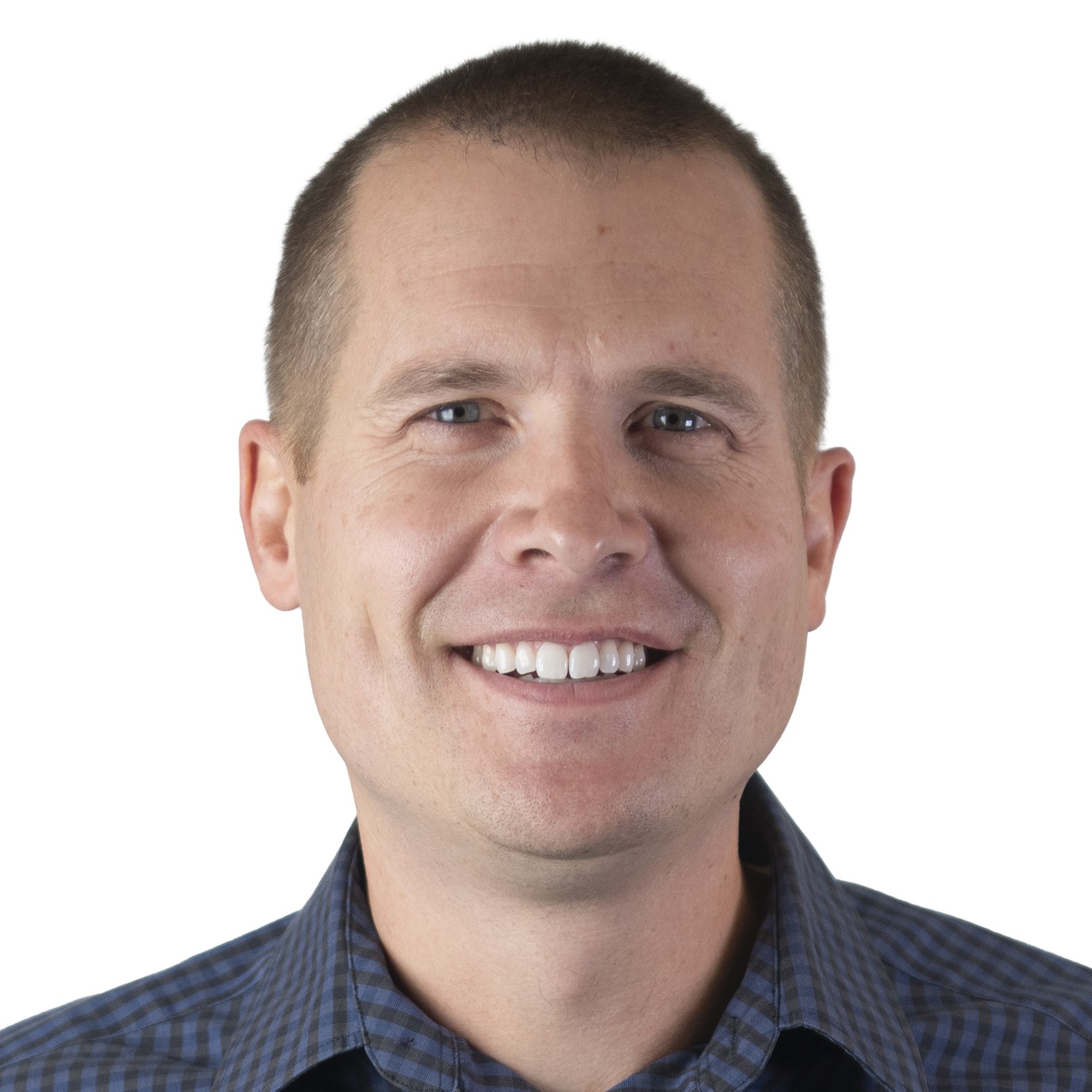
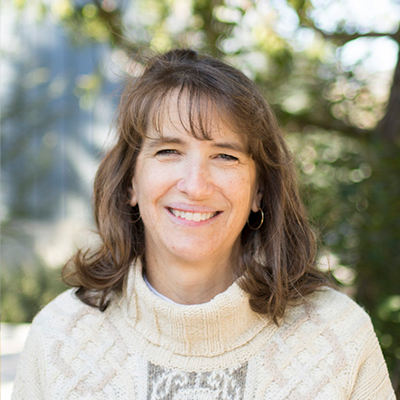
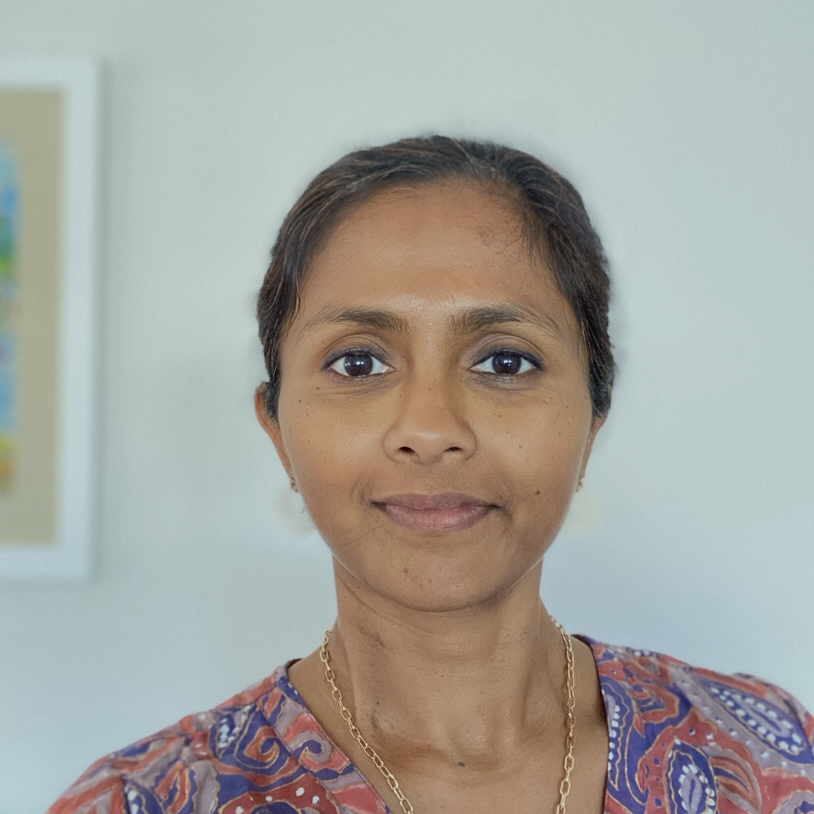
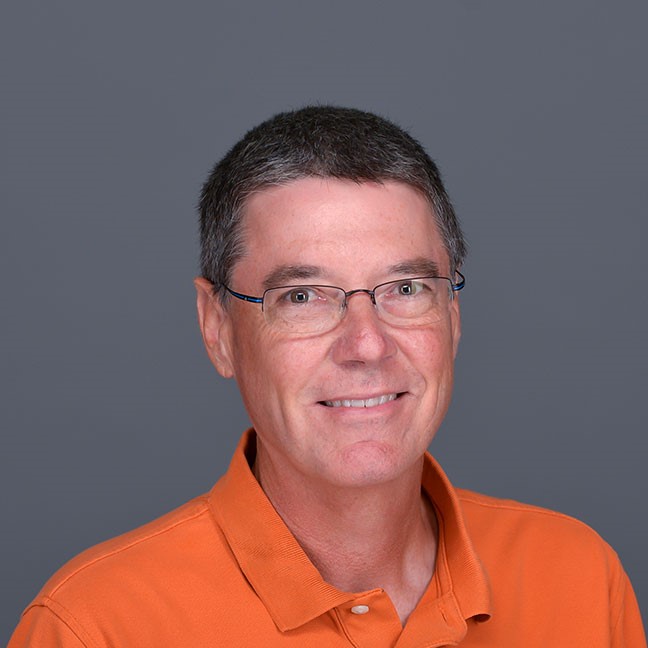
Nathan Tintle (University of Illinois - Chicago), Beth Chance + Soma Roy + Karen McGaughey (Cal Poly - San Luis Obispo), Todd Swanson (Hope College)
Abstract
Increasingly, introductory and intermediate statistics course enrollments are threatened by the data science movement. Students, faculty colleagues, and institutions are, rightly, questioning whether students are better served by a ‘course in data science.’ Furthermore, these same individuals (and maybe you as well!) are asking how to modify your course content in light of changes in the way big data and data science are impacting statistical practice. What should you do? Do some of your current practices qualify? How do we let others know?
In this breakout session, we’ll explore ways that many of us are already teaching some of the core ‘topics in data science’ in our algebra-based introductory statistics courses—and might not even know it. Topics like data provenance, data visualization, data ethics, and data communication (among others) are major themes in data science and are conversations you are probably already having in your introductory statistics course. In this session we’ll take a standard, algebra-based, GAISE compliant introductory statistics course and map its learning objectives/content onto data science learning objectives. We’ll also see a few small things you could add to your course to make it even more ‘data sciency’ than it already is.