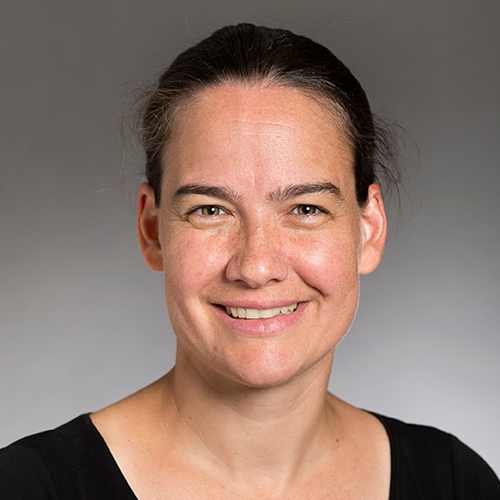
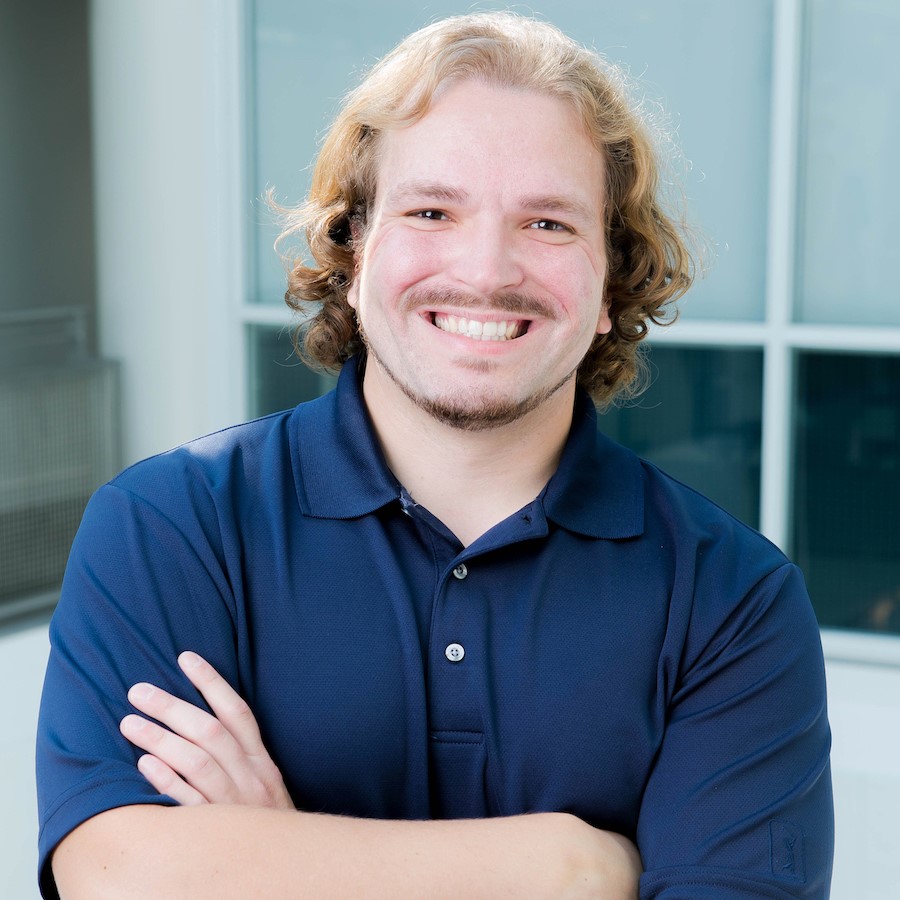
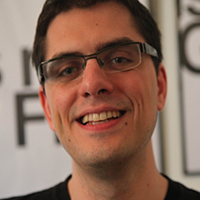
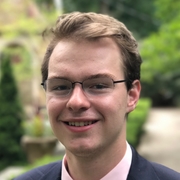
Rebecca Nugent, Ron Yurko, Philipp Burckhardt, & Francis Kovacs (Carnegie Mellon University)
Abstract
With the growing emphasis on understanding reproducibility and replicability, how do we illustrate these concepts to introductory level students? Moreover, given subjective choices (e.g. graphs, transformations) and differing student perspectives, there are many possible data analysis workflows rarely converging on one true answer. Students need early and sustained exposure to how data analysis decisions can impact results while learning best practices for reproducing work. Mimicking the "Many Analysts, One Data Set" crowd-sourcing paper by Silberzahn et al. (2015, 2018), participants will interact with data looking for evidence of racial bias in World Cup red cards, using the Carnegie Mellon Integrated Statistics Learning Environment’s data analytics and report-writing tool that tracks data analysis workflows and offers functionality for collaboration such as peer review. In this session, we discuss factors that may impact data analysis reproducibility, related classroom exercises, and ideas for how to support the entire introductory data analysis pipeline.