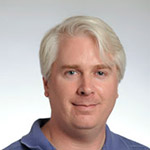
Peter E. Freeman (Carnegie Mellon University)
Abstract
Undergraduate statistics students at Carnegie Mellon crave the opportunity to apply what they have learned in their classes to the analysis of data. However, the sheer number of students in our major currently precludes us from being able to provide those opportunities to all who want them. In this webinar, I discuss a framework for introducing early undergraduates to statistical research methodology--the procedures by which statisticians go about approaching and analyzing data--that I have developed to help rectify this situation. In this framework, students are provided data and are guided through analyses, while being taught basic concepts of statistical learning that they have not necessarily been exposed to in lecture-based classes: inference vs. prediction, supervised vs. unsupervised learning, data splitting, etc. I specifically target early undergraduates because doing so has several tangible benefits, including that (a) it is never too early to impart sound statistical thinking to students, students who may be facing real-world data for the first time, (b) it provides an excellent opportunity to demystify algorithms (e.g., random forest) and practices (e.g., data splitting) to which students will eventually be (re-)exposed in their advanced classes, and (c) demonstrated experience in data analysis helps open doors for early undergraduates that otherwise might remain closed (e.g., summer internships, more advanced research opportunities with professors, etc.).