Sandbox: Difference between revisions
Line 1: | Line 1: | ||
==Bogus statistics== | ==Bogus statistics== | ||
[How To Spot a Bogus Statistic]<br> | [How To Spot a Bogus Statistic]<br> |
Revision as of 20:37, 24 July 2015
Bogus statistics
[How To Spot a Bogus Statistic]
by Geoffrey James, Inc.com, 30 May 2015
The article begins by citing Bill Gates recent recommendation that everyone should read the Darrell Huff classic How to Lie With Statistics.
As an object lesson, James considers efforts to dispute the scientific consensus on anthropogenic climate change.
Submitted by Bill Peterson
Predicting GOP debate participants
Ethan Brown posted this following link on the Isolated Statisticians list:
- The first G.O.P. debate: Who’s in, who’s out and the role of chance
- by Kevin Quealy and Amanda Cox , "Upshot" blog New York Times, 21 July 2015
Sleeping beauties
Douglas Rogers sent a link to the following:
- Defining and identifying Sleeping Beauties in science
- by Qing Ke, et. al., PNAS (vol. 112 no. 24), 2015.
A "sleeping beauty" is an article one that achieves few if any citations in the years immediately following its publication, but then sees a large burst of interest many years later. The PNAS paper derives a so-called "beauty coefficient", denoted B, that depends on how many years the paper is dormant and how many citations it ultimately receives.
The PNAS paper is quite technical, but a popular account can be found here:
- The sleeping beauties of science
- by Nathan Collins, Pacific Standard, 28 May 2015
This article cites a 1901 paper by Karl Person, On lines and planes of closest fit to systems of points in space, as a classic sleeping beauty. It was not widely cited until 2002!
Some math doodles
<math>P \left({A_1 \cup A_2}\right) = P\left({A_1}\right) + P\left({A_2}\right) -P \left({A_1 \cap A_2}\right)</math>
<math>\hat{p}(H|H)</math>
Accidental insights
My collective understanding of Power Laws would fit beneath the shallow end of the long tail. Curiosity, however, easily fills the fat end. I long have been intrigued by the concept and the surprisingly common appearance of power laws in varied natural, social and organizational dynamics. But, am I just seeing a statistical novelty or is there meaning and utility in Power Law relationships? Here’s a case in point.
While carrying a pair of 10 lb. hand weights one, by chance, slipped from my grasp and fell onto a piece of ceramic tile I had left on the carpeted floor. The fractured tile was inconsequential, meant for the trash.
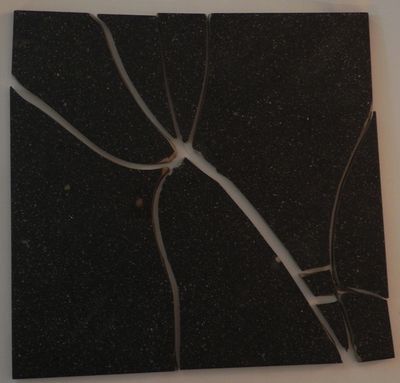
As I stared, slightly annoyed, at the mess, a favorite maxim of the Greek philosopher, Epictetus, came to mind: “On the occasion of every accident that befalls you, turn to yourself and ask what power you have to put it to use.” Could this array of large and small polygons form a Power Law? With curiosity piqued, I collected all the fragments and measured the area of each piece.
Piece | Sq. Inches | % of Total |
---|---|---|
1 | 43.25 | 31.9% |
2 | 35.25 | 26.0% |
3 | 23.25 | 17.2% |
4 | 14.10 | 10.4% |
5 | 7.10 | 5.2% |
6 | 4.70 | 3.5% |
7 | 3.60 | 2.7% |
8 | 3.03 | 2.2% |
9 | 0.66 | 0.5% |
10 | 0.61 | 0.5% |
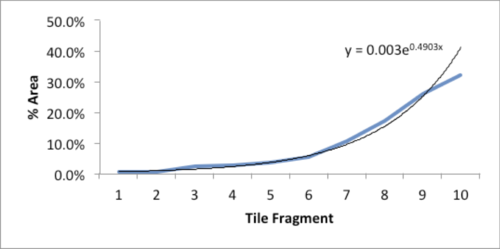
The data and plot look like a Power Law distribution. The first plot is an exponential fit of percent total area. The second plot is same data on a log normal format. Clue: Ok, data fits a straight line. I found myself again in the shallow end of the knowledge curve. Does the data reflect a Power Law or something else, and if it does what does it reflect? What insights can I gain from this accident? Favorite maxims of Epictetus and Pasteur echoed in my head: “On the occasion of every accident that befalls you, remember to turn to yourself and inquire what power you have to turn it to use” and “Chance favors only the prepared mind.”
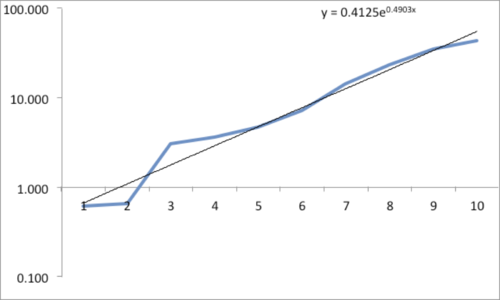
My “prepared” mind searched for answers, leading me down varied learning paths. Tapping the power of networks, I dropped a note to Chance News editor Bill Peterson. His quick web search surfaced a story from Nature News on research by Hans Herrmann, et. al. Shattered eggs reveal secrets of explosions. As described there, researchers have found power-law relationships for the fragments produced by shattering a pane of glass or breaking a solid object, such as a stone. Seems there is a science underpinning how things break and explode; potentially useful in Forensic reconstructions. Bill also provided a link to a vignette from CRAN describing a maximum likelihood procedure for fitting a Power Law relationship. I am now learning my way through that.
Submitted by William Montante