Chance News 15: Difference between revisions
(35 intermediate revisions by 3 users not shown) | |||
Line 9: | Line 9: | ||
From the February 2006 RSS news we have: | From the February 2006 RSS news we have: | ||
<blockquote> One primary school in East London has a catchment area of 110 metres. | <blockquote> One primary school in East London<br> has a catchment area of 110 metres. | ||
<div align="right"> ''Sunday Telegraph''<br> | <div align="right"> ''Sunday Telegraph''<br> | ||
23 October 2005</div></blockquote> | 23 October 2005</div></blockquote> | ||
And from the March 2006 RSS news we have: | |||
<blockquote> Fewer names appear in the top 100<br> than ten years ago.<br><br> | |||
(from report on most popular names<br> given to babies born in Scotland in 2005) | |||
<div align="right"> ''The Scotsman''<br> | |||
24 December 2005</div></blockquote> | |||
==More generous than they realize== | |||
From the novel Summer Harbor, by Susan Wilson (NY: ATRIA Books, 2003): | |||
<blockquote> The waitress coyly asked if they wanted change of the two twenties Kiley laid down on a thirty-two-dollar tab.<br>"No, we’re fine."<br>"That’s a twenty percent tip, Mom."<br>"I’m feeling generous."</blockquote> | |||
Submitted by Margaret Cibes | |||
==More on medical studies that conflict with previous studies== | ==More on medical studies that conflict with previous studies== | ||
Line 44: | Line 58: | ||
==Economists analyze the tv show "Deal or No Deal?"== | ==Economists analyze the tv show "Deal or No Deal?"== | ||
[http://www.post-gazette.com/pg/06012/636981.stm Why game shows have | [http://www.post-gazette.com/pg/06012/636981.stm Why game shows have economists glued to their TVs].<br> | ||
''Wall Street Journal'', Jan. 12, 2006<br> | ''Wall Street Journal'', Jan. 12, 2006<br> | ||
Charles Forelle | Charles Forelle | ||
[http://www.npr.org/templates/story/story.php?storyId=5244516 Economists Learn from Game Show | [http://www.npr.org/templates/story/story.php?storyId=5244516 Economists Learn from Game Show "Deal or No Deal'].<br> | ||
NPR, March 3, 2006, All | NPR, March 3, 2006, ''All Things Considered'' <br> | ||
David Kestenbaum | David Kestenbaum | ||
Line 68: | Line 82: | ||
* The banker makes one last offer; the contestant accepts that offer or takes whatever money is in the initially chosen briefcase. | * The banker makes one last offer; the contestant accepts that offer or takes whatever money is in the initially chosen briefcase. | ||
So the banker is always trying to buy out the player. If he fails the player will end up with the amount in his | So the banker is always trying to buy out the player. If he fails, the player will end up with the amount in his briefcase. | ||
The best way to understand the game is to play it [http://www.nbc.com/Deal_or_No_Deal/game/ here ] on the NBC website. | The best way to understand the game is to play it [http://www.nbc.com/Deal_or_No_Deal/game/ here ] on the NBC website. | ||
We did this playing the only the first round. We chose number 13 for our briefcase. We | We did this, playing the only the first round. We chose number 13 for our briefcase. We were then asked to choose six briefcases. We chose briefcases 3,10,12,13,19, 25. Here is the result of this round: | ||
<center>[[Image:deal.jpg|400px|After the first round.]] | <center>[[Image:deal.jpg|400px|After the first round.]] | ||
</center> | </center> | ||
Our six choices eliminated the amounts 1, 50, 75, 300, 400,000, 500,000. The expected value of our | Our six choices eliminated the amounts 1, 50, 75, 300, 400,000, 500,000. The expected value of our briefcase was now the average of the amounts in the remaining briefcases. Making this calculation we found that this is $125,900 (rounded to the nearest dollar). The banker offered us $29,854 to quit. So we would have to have been pretty risk adverse to accept the banker's offer at this point. | ||
In order to see if the banker's offers get better, we played a second game in which we refused all the banker's offers. With this strategy we | In order to see if the banker's offers get better as we pick more briefcases, we played a second game in which we refused all the banker's offers. With this strategy we started with an expected winning given by the average of the initial amounts, which we found to be $131,478. | ||
We then played all 9 rounds. In each round we recalculated the expected amount in our briefcase and recorded the banker's offer but refused it. Here are the results: | We then played all 9 rounds. In each round we recalculated the expected amount in our briefcase and recorded the banker's offer but refused it. Here are the results: | ||
<center><table width=" | <center> | ||
<table width="53%" border="1"> | |||
<tr> | |||
<td width="18%"><div align="center">Round</div></td> | |||
<td width="30%"><div align="center">Expected Value</div></td> | |||
<td width="29%"><div align="center">Offer</div></td> | |||
<td width="29%"><div align="center">Percent of Expected Value</div></td> | |||
</tr> | |||
<tr> | |||
<td><div align="center">1</div></td> | |||
<td><div align="center">130,857</div></td> | |||
<td><div align="center">30,565</div></td> | |||
<td><div align="center">23</div></td> | |||
</tr> | |||
<tr> | |||
<td><div align="center">2</div></td> | |||
<td><div align="center">142,475</div></td> | |||
<td><div align="center">37,361</div></td> | |||
<td><div align="center">26</div></td> | |||
</tr> | |||
<tr> | |||
<td><div align="center">3</div></td> | |||
<td><div align="center">125,186</div></td> | |||
<td><div align="center">54,507</div></td> | |||
<td><div align="center">44</div></td> | |||
</tr> | |||
<tr> | |||
<td><div align="center">4</div></td> | |||
<td><div align="center">153,319</div></td> | |||
<td><div align="center">57,554</div></td> | |||
<td><div align="center">38</div></td> | |||
</tr> | |||
<tr> | |||
<td><div align="center">5</div></td> | |||
<td><div align="center">170,967</div></td> | |||
<td><div align="center">67,160</div></td> | |||
<td><div align="center">39</div></td> | |||
</tr> | |||
<tr> | |||
<td><div align="center">6</div></td> | |||
<td><div align="center">205,160</div></td> | |||
<td><div align="center">108,950</div></td> | |||
<td><div align="center">53</div></td> | |||
</tr> | |||
<tr> | |||
<td><div align="center">7</div></td> | |||
<td><div align="center">256,425</div></td> | |||
<td><div align="center">176,781</div></td> | |||
<td><div align="center">69</div></td> | |||
</tr> | |||
<tr> | |||
</table> | <td><div align="center">8</div></td> | ||
<td><div align="center">341,800</div></td> | |||
<td><div align="center">317,700</div></td> | |||
<td><div align="center">93</div></td> | |||
</tr> | |||
<tr> | |||
<td><div align="center">9</div></td> | |||
<td><div align="center">512,500</div></td> | |||
<td><div align="center">367,500</div></td> | |||
<td><div align="center">72</div></td> | |||
</tr> | |||
</table> | |||
</center> | </center> | ||
We note that the banker's offer at each round | We note that the banker's offer at each round was less than the expected amount in our briefcase. While it seemed clear at the beginning we would be right to refuse the offer, as we get nearer the end this is not so obvious. For example, after the 8th round the only amounts available were $400, $25,000, and $1,000,000. We were offered $317,700, a pretty nice amount of money. If we rejected it we would have a 2/3 chance of ending up with a relatively small amount and a 1/3 chance of getting the million dollars. We might well be sufficiently risk averse at this point to accept the offer. However, we didn't and the briefcase we chose had the $400. Now the only amounts left were $25,000 and $1,000,000. We were now offered $367,500. Again we might have thought twice about refusing this. But we did refuse it and we opened the last briefcase, which had the million dollars, So our briefcase had $25,000. If you watch the TV program you will find that most contestants refuse the banker's offers in the early rounds but accept it in one of the later rounds. | ||
“Deal or No Deal” was introduced in Australia in 2003 and currently airs in 38 countries including the U.K. Those who feel that Excel is slow to fix its statistical errors will be amused by the Wikipedia [http://en.wikipedia.org/wiki/Deal_or_No_Deal_(UK) account] (See Predictable Sequence Controversy) of what happened to the UK “Deal no No Deal” game when the Excel random number generator was not properly seeded. Apparently the Excel error allowed one to estimate the contents of the remaining boxes--obviously very useful information. | |||
This error was first reported by the U.K. Bother's Bar website. You can see the response of the “Deal or No Deal” producers of their [http://www.bothersbar.co.uk/ home page] (see Glenn Hugill writes). You can find an interesting discussion of the U.K. version of Deal or no Deal by the Brother's Bar [http://www.bothersbar.co.uk/dealexplanation.htm here.] In particular in their FAQ they give the following answer to question everyone asks: How does the Banker come up with the offers? | |||
<blockquote> Banker's software throws up a lower and higher cash sum based on the values left on the board. The Banker then puts his own spin on the proceedings by picking a figure between the two boundaries. Factors such as the attitude of the player and who is having the better run of luck are all considerations. Producer Glenn Hugill says "I don't pretend to know how he operates but I believe there is a range which is considered to mathematically cover the financial risk. However if the Banker wishes to gamble or indeed play safe he can accordingly pitch his offer anywhere below or above it." </blockquote> | |||
Bother's Bar also collected [http://www.bothersbar.co.uk/dondstats.htm data] which made it possible to compare the Box(Briefcase) value with the Banker's offer. You will find here the Box(briefcase) value, Peak bank offer, and Prize won for all programs from October 31 2005 to the present time. | |||
For their Economics paper, Post and his co-authors obtained videos of 53 episodes from Australia and the Netherlands. From these they determined, for each contestant and each round, the situation the contestant faced in terms of the amounts of money in the remaining briefcases, the Banker's offer and their decision to accept or refuse the offer. The authors then analyze this data using a measure of relative risk aversion developed by Arrow and Pratt (RRA). They write: | |||
<blockquote>In every game round, a unique RRA coefficient can be determined at which the contestant would be indifferent between accepting and rejecting the bank offer. If the contestant accepts the offer, his RRA must be higher than this value; if the offer is rejected, his RRA must be lower.</blockquote> | <blockquote>In every game round, a unique RRA coefficient can be determined at which the contestant would be indifferent between accepting and rejecting the bank offer. If the contestant accepts the offer, his RRA must be higher than this value; if the offer is rejected, his RRA must be lower.</blockquote> | ||
Line 145: | Line 178: | ||
They also investigate how this RRA changes according to other variables such as estimated income and education. | They also investigate how this RRA changes according to other variables such as estimated income and education. | ||
The authors find that, for a wealth level of '''€'''25,000, an average over all | The authors find that, for a wealth level of '''€'''25,000, an average over all these contestants gives an RRA 1.61. This estimate decreases when the wealth level decreases and increases when it increases. The degree of risk aversion differs strongly across the contestants, some exhibiting strong risk adverse behavior (RRA > 5) and others risk-seeking behavior (RRA < 0). They say: | ||
<blockquote>The degree of risk aversion differs strongly across the contestants, some exhibiting strong risk averse behavior (RRA > 5) and others risk seeking behavior (RRA< 0). The differences can be explained in large part by the earlier outcomes experienced by the contestants in previous rounds of the game. Most notably, RRA generally decreases following losses. Contestants facing a large reduction in the expected prize during the game even exhibit risk seeking behavior. </blockquote> | <blockquote>The degree of risk aversion differs strongly across the contestants, some exhibiting strong risk averse behavior (RRA > 5) and others risk seeking behavior (RRA< 0). The differences can be explained in large part by the earlier outcomes experienced by the contestants in previous rounds of the game. Most notably, RRA generally decreases following losses. Contestants facing a large reduction in the expected prize during the game even exhibit risk-seeking behavior. </blockquote> | ||
===DISCUSSION=== | ===DISCUSSION=== | ||
(1) Suppose that at each round the banker offered you the expected value of the amount in your briefcase and you were only interested in maximizing your expected winning. Would it matter when you stopped? | (1) Suppose that at each round the banker offered you the expected value of the amount in your briefcase and you were only interested in maximizing your expected winning. Would it matter when you stopped? | ||
(2) | (2) Professor Post tells us: | ||
<blockquote>The banker behaved in a predictable manner, with offers around 5-10% of expected value in round one and 100% in the later rounds. Also, for losers, the offers are relatively generous, sometimes exceeding 100%.</blockquote> | |||
This leaves many questions about how the banker made his offers: Is there a real Banker or is it all done by an algorithm? The Bother's Bar’s comments suggest there is a real Banker for the U.K. program. Does the offer depend only on the expected amount in a player's briefcase? If you are teaching a probability or statistics course you could ask the students to look into one of these questions or to make their own hypothesis about how the offers are made. | |||
(3) Suppose you knew how the offer is determined. How would this affect your strategy for playing the game? | |||
(4) Suppose you are told that the banker knows what is in your briefcase. How would this affect the way you played the game? | |||
(5) Using the Bother's Bar [http://www.bothersbar.co.uk/dondstats.htm data], compare the sum of the prizes given out with the sum of the Box values when the prizes are awarded. | |||
Submitted by Laurie Snell | |||
==Sexual prediction== | ==Sexual prediction== | ||
Line 197: | Line 236: | ||
In Minnesota, according to James Walsh of the ''Minneapolis Star Tribune'' ([http://www.startribune.com/1592/story/286907.html March 6, 2006]), the trick is to have the low-scoring students take a different test. "To some, it may look like we're gaming the system" says Tim Vansickle, the Minnesota director of assessments. Minnesota does have many students whose native language is not English and Vansickle denies that the alternative test is not meant to help schools keep off "the list" of schools deemed failing. | In Minnesota, according to James Walsh of the ''Minneapolis Star Tribune'' ([http://www.startribune.com/1592/story/286907.html March 6, 2006]), the trick is to have the low-scoring students take a different test. "To some, it may look like we're gaming the system" says Tim Vansickle, the Minnesota director of assessments. Minnesota does have many students whose native language is not English and Vansickle denies that the alternative test is not meant to help schools keep off "the list" of schools deemed failing. | ||
Another technique according to Jay Mathews of the Washington Post (February 28, 2006), is to engage in strange arithmetic. He quotes Monte Dawson, the testing and assessment director for Alexandria, Virginia: "Remediation Recovery, which has been around since 2001, means that fourth grade students who failed the third grade test in 2004, got to retake the third grade test in 2005. Up until this year (2005), if they passed the third grade test, then they were included in the numerator only of the calculation to determine the third grade passing score. As illustration, if 4 out of 5 third grade students passed and 1 out of 5 fourth grade Remediation Recovery students passed, the passing percentage would be 100 percent." | Another technique according to Jay Mathews of the Washington Post ([http://www.washingtonpost.com/wp-dyn/content/article/2006/02/28/AR2006022800519.html February 28, 2006]), is to engage in strange arithmetic. He quotes Monte Dawson, the testing and assessment director for Alexandria, Virginia: "Remediation Recovery, which has been around since 2001, means that fourth grade students who failed the third grade test in 2004, got to retake the third grade test in 2005. Up until this year (2005), if they passed the third grade test, then they were included in the numerator only of the calculation to determine the third grade passing score. As illustration, if 4 out of 5 third grade students passed and 1 out of 5 fourth grade Remediation Recovery students passed, the passing percentage would be 100 percent." | ||
Mathews, the ''Washington Post's'' education columnist, is puzzled by this explanation so he leaves it to the spokesman for the Virginia Department of Education, Charles Pyle. Mathews translates Pyle's remarks thusly: "in 2000, the state school board changed the counting procedure to encourage more schools to do what Maury [the school featured in the article] did -- give the students who failed some extra help and let them try again. Often the second-test passing rates of students who flunk a test initially are lower than their class's overall passing rate, since they are the class's weakest students. So if those second-test results were combined with the first test results in the usual way, it would likely lower the overall percentage and make the school look worse than otherwise. School districts in Virginia figured this out and resisted the urge to work with their lowest-performing students and test them again." | Mathews, the ''Washington Post's'' education columnist, is puzzled by this explanation so he leaves it to the spokesman for the Virginia Department of Education, Charles Pyle. Mathews translates Pyle's remarks thusly: "in 2000, the state school board changed the counting procedure to encourage more schools to do what Maury [the school featured in the article] did -- give the students who failed some extra help and let them try again. Often the second-test passing rates of students who flunk a test initially are lower than their class's overall passing rate, since they are the class's weakest students. So if those second-test results were combined with the first test results in the usual way, it would likely lower the overall percentage and make the school look worse than otherwise. School districts in Virginia figured this out and resisted the urge to work with their lowest-performing students and test them again." | ||
Line 224: | Line 263: | ||
Joe Nocere | Joe Nocere | ||
Michael Mauboussin is a well known Wall Street strategist who also is an adjunct professor at Columbia Business School. Every year since 1993 he has asked his students to vote for the winners in 12 | Michael Mauboussin is a well known Wall Street strategist who also is an adjunct professor at Columbia Business School. Every year since 1993 he has asked his students to vote for the winners in 12 categories including major and relatively obscure contests. We read: | ||
<blockquote>This year, the pick that got the most votes — the consensus pick, he calls it — turned out to be right in 9 of the 12 categories, including, amazingly enough, film editing and art direction. And yet, of the 47 students who participated, only one matched the accuracy of the consensus. None did better, and most did much worse; according to Mr. Mauboussin, the average number of correct answers per ballot this year was only 4.1. "It has never failed," he said. "The consensus invariably does much better than the average student." </blockquote> | <blockquote>This year, the pick that got the most votes — the consensus pick, he calls it — turned out to be right in 9 of the 12 categories, including, amazingly enough, film editing and art direction. And yet, of the 47 students who participated, only one matched the accuracy of the consensus. None did better, and most did much worse; according to Mr. Mauboussin, the average number of correct answers per ballot this year was only 4.1. "It has never failed," he said. "The consensus invariably does much better than the average student." </blockquote> | ||
The article also discusses other evidence that consensus predictions are often better than individual predictions such as the [http://www.biz.uiowa.edu/iem/ Iowa Electronic Market] ([http://www.dartmouth.edu/~chance/chance_news/recent_news/chance_news_13.06.html#item17 Chance News 13.06] , Holywood Stock Exchange, and a new one | The article also discusses other evidence that consensus predictions are often better than individual predictions such as the [http://www.biz.uiowa.edu/iem/ Iowa Electronic Market] ([http://www.dartmouth.edu/~chance/chance_news/recent_news/chance_news_13.06.html#item17 (Chance News 13.06)] , [http://www.hsx.com/ Holywood Stock Exchange] [http://www.dartmouth.edu/~chance/chance_news/recent_news/chance_news_12.02.html#item5 (Chance News 12.02)], and a new one [http://www.hedgestreet.com HedgeStreet] whose motto is: "It's your economy. Trade it." | ||
===DISCUSSION=== | |||
(1) Choose one of the 12 categories and assume that there are 5 nominees for this category labeled 1,2,3,4,5. Assume that each of the 47 students rolls a five-sided die to determine their prediction. What is the probability that that their consensus vote is the Oscar winner for this category? | |||
(2) Assume that there are 5 contestants for each of the 12 categories and the 47 students guess the winner in each category. What is the probability that their consensus vote is the Oscar winner in 9 or more of the 12 categories? | |||
==Science in 2020 – databases and statistics== | |||
[http://www.economist.com/science/displayStory.cfm?story_id=5655067 Computing the future], The Economist, 25th March 2006<br> | |||
[http://www.nature.com/nature/focus/futurecomputing/index.html 2020 – Future of Computing], Nature, 23rd February 2006.<br> | |||
[http://www.nature.com/nature/journal/v440/n7083/pdf/440409a.pdf Exceeding human limits], Stephen H. Muggleton, Department of Computing and the Centre for Integrative | |||
Systems Biology at Imperial College London.<br> | |||
This week’s <em>Nature</em> has a series of news features and commentaries looking at how tomorrow’s computer technology will change the face of science. | |||
For example, in [http://www.nature.com/nature/journal/v440/n7083/pdf/440413a.pdf Science in an exponential world], Alexander Szalay in the Department of Physics | |||
and Astronomy, Johns Hopkins University and Jim Gray at Microsoft Research, San Francisco, | |||
promote the use of database tools, such as ‘data cubes’, | |||
to ‘see’ a new pattern or find a | |||
data point that does not fit a hypothesis. | |||
The authors claim that many scientists | |||
now ‘mine’ available databases, looking for new patterns and discoveries, without ever | |||
picking up a pipette. | |||
So the authors warn about a forthcoming data explosion: | |||
<blockquote> | |||
computers also perform data analysis; Matlab, Mathematica | |||
and Excel are popular analysis tools. But none | |||
of these programs scale up to handle millions | |||
of data records — and they are primitive by | |||
most standards. As data volumes grow, it is | |||
increasingly arduous to extract knowledge. | |||
Scientists must labour to organize, sort and | |||
reduce the data, with each analysis step producing | |||
smaller data sets that eventually lead to | |||
the big picture. | |||
</blockquote> | |||
Their two-page paper also discusses how | |||
scientists are increasingly analysing complex | |||
systems that require data to be combined | |||
from several groups and even several disciplines. | |||
<blockquote> | |||
Data is shared across departments and time zones, and | |||
important discoveries are made by scientists | |||
and teams who combine different skill sets — | |||
not just biologists, physicists and chemists, | |||
but also computer scientists, statisticians and | |||
data-visualization experts. | |||
</blockquote> | |||
This leads the authors to conclude that today’s graduate students need | |||
formal training in areas beyond their central | |||
discipline, such as data management, | |||
computational concepts and statistical techniques. | |||
On the topic of using databases to handle the data deluge, they say: | |||
<blockquote> | |||
the systematic use of databases | |||
has become an integral part of the scientific | |||
process. Databases provide tools to organize | |||
large data sets, find objects that match certain | |||
criteria, compute statistics about the data, and | |||
analyse them to find patterns. Many experiments | |||
today load their data into databases | |||
before attempting to analyse them. But there | |||
are few tools to properly visualize data across | |||
multiple scales and data sets. If we can no | |||
longer examine all the data on a single piece of | |||
paper, how can we ‘see’ a new pattern or find a | |||
data point that does not fit a hypothesis? | |||
</blockquote> | |||
Another issue that is touched on is the reproducibility of any analysis. | |||
With experiments involving more people and data, | |||
documenting the data, software versions, models, parameter settings etc | |||
is now becoming a task best left to computers to record. | |||
But for the analysis to be repeatable in twenty years’ time | |||
the tools as well as the data will have to be archieved. | |||
In another Nature commentary, | |||
Stephen Muggleton, the head of computational bio-informatics at Imperial College, London, claims that computers will soon play a role in formulating scientific hypotheses and designing and running experiments to test them. The data deluge is such that human beings can no longer be expected to spot patterns in the data. | |||
So the future of science involves the | |||
expansion of automation in all its aspects | |||
including data collection, hypothesis formation and experimentation. | |||
For example, machine-learning techniques | |||
from computer science (including neural nets | |||
and genetic algorithms) are being used to | |||
automate the generation of scientific hypotheses | |||
from data. Some of the | |||
more advanced forms of | |||
machine learning enable new | |||
hypotheses, in the form of logical | |||
rules and principles, to be | |||
extracted relative to predefined | |||
background knowledge. | |||
One such development that Muggleton mentions | |||
is the emergence within computer science | |||
of new formalisms that integrate two major branches of mathematics: | |||
mathematical logic and probability calculus. | |||
<blockquote> | |||
Mathematical logic provides a formal | |||
foundation for logic programming languages; | |||
probability calculus provides the basic axioms of probability for | |||
statistical models, such as bayesian networks. | |||
The resulting ‘probabilistic logic’ is a formal | |||
language that supports statements of sound | |||
inference, such as ‘The probability of A being | |||
true if B is true is 0.7’. | |||
Although it is early days, such research holds | |||
the promise of sound integration of scientific | |||
models from the statistical and computer-science | |||
communities. | |||
</blockquote> | |||
Muggleton also mentions the growing trend | |||
of automatically feeding the results an experiment | |||
back into the model to improve the final results. | |||
<blockquote> | |||
laboratory robots conducted experiments | |||
on yeast using a process known as ‘active learning’. | |||
The aim was to determine the function of | |||
several gene knockouts by varying the quantities | |||
of nutrient provided to the yeast. The | |||
robot used a form of inductive logic programming | |||
to select experiments that would | |||
discriminate between contending hypotheses. | |||
Feedback on each experiment was provided | |||
by data reporting yeast survival or death. | |||
The robot strategy that worked best | |||
(lowest cost for a given accuracy of prediction) | |||
not only outperformed two other automated | |||
strategies, based on cost and | |||
random-experiment selection, but also outperformed | |||
humans given the same task. | |||
</blockquote> | |||
===Questions=== | |||
* How realistic is it to expect to “examine all the data on a single piece of paper”? | |||
* The authors call for better visualisation tools but don’t mention any examples, either good or bad. Can you suggest reasons why they might have chosen to side-step this issue. | |||
===Further reading=== | |||
* The full suite of Nature articles are [http://www.nature.com/nature/focus/futurecomputing/index.html#research available on line] | |||
* Visit [http://blogs.nature.com/news/blog/2006/03/2020_computing.html News@nature.com's newsblog] to read and post comments on the future of computing. | |||
* [http://research.microsoft.com/towards2020science Microsoft 2020 Science website] | |||
Submitted by John Gavin. |
Latest revision as of 19:33, 14 May 2006
Quotation
Take statistics. Sorry, but you'll find later in life that it's handy to know what a standard deviation is.
David Brooks
New York Times, March 2, 2006
This appears on a list of core knowledge that Brooks says will be sufficient to give you a great education even if you don't make Harvard.
Forsooth
From the February 2006 RSS news we have:
One primary school in East London
has a catchment area of 110 metres.Sunday Telegraph
23 October 2005
And from the March 2006 RSS news we have:
Fewer names appear in the top 100
than ten years ago.
(from report on most popular names
given to babies born in Scotland in 2005)The Scotsman
24 December 2005
More generous than they realize
From the novel Summer Harbor, by Susan Wilson (NY: ATRIA Books, 2003):
The waitress coyly asked if they wanted change of the two twenties Kiley laid down on a thirty-two-dollar tab.
"No, we’re fine."
"That’s a twenty percent tip, Mom."
"I’m feeling generous."
Submitted by Margaret Cibes
More on medical studies that conflict with previous studies
Humans, being what they are, it is only natural that when a study's consequences seem plausible there is no need to look too closely. On the other hand, when the outcomes go against what was expected, a great deal of inspection is called for. This was discussed here.
The Wall Street Journal of February 28, 2006 details possible reasons for why the Women's Health Initiative might have had design flaws leading to "murky results." In summary, the WSJ reported:
- Calcium/Vitamin D study
- Message: Supplements don't protect bones or cut risk of colorectal cancer.
- Problem: Those in placebo group also took supplements in many cases.
- Message: Supplements don't protect bones or cut risk of colorectal cancer.
- Low-fat diet study:
- Message: Doesn't cut risk of breast cancer.
- Problem: Few met the fat goal.
- A 22% drop in risk for women who cut fat the most got little emphasis.
- Message: Doesn't cut risk of breast cancer.
- Hormone study:
- Message: No benefit, possible increased cancer and heart risk.
- Problem: Most in study were too old for this to apply to menopausal women.
- Message: No benefit, possible increased cancer and heart risk.
More generally, according to the WSJ, "Design problems in all of the trials mean the results don't really answer the questions they were supposed to address. And a flawed communications effort led to widespread misinterpretation of results by the news media and public."
In particular, in order to reduce the number of participants for the studies, "more than half [of the women] took part in at least two of them, and more that 5,000 were in all three trials." As might be imagined, "Among problems this posed was simple burnout" which "contributed to compliance problems that plagued all three and hurt the reliability of their results."
Another problem was the difficulty of double blinding for the hormone study since any hot flashes would indicate to the patient (and to her physician) that she was in the placebo arm; to get around this impediment, the vast majority of the women recruited were well past menopause, thus biasing the results against the benefits of hormone replacement.
So where are we after 68,132 female participants, "fifteen years and $725 million later"? More than likely, the Women's Health Initiative study will be in and out of the news for some time to come because of its ambiguity.
Submitted by Paul Alper
Economists analyze the tv show "Deal or No Deal?"
Why game shows have economists glued to their TVs.
Wall Street Journal, Jan. 12, 2006
Charles Forelle
Economists Learn from Game Show "Deal or No Deal'.
NPR, March 3, 2006, All Things Considered
David Kestenbaum
Deal or No Deal? Decision making under risk in a large-payoff game show
Thierry Post, Martijn Van Den Assem, Guido Baltussen, Richard H. Thaler
February 2006
The authors of the research paper write:
The popular television game show "Deal or No Deal" offers a unique opportunity for analyzing decision making under risk: it involves very large and wide-ranging stakes, simple stop-go decisions that require minimal skill, knowledge or strategy and near-certainty about the probability distribution.
Here is a nice description of the game from the MS Math in the Media magazine:
- Twenty-six known amounts of money, ranging from one cent to one million dollars, are (symbolically) randomly placed in 26 numbered, sealed briefcases. The contestant chooses a briefcase. The unknown sum in the briefcase is the contestant's.
- In the first round of play, the contestant chooses 6 of the remaining 25 briefcases to open. Then the "banker" offers to buy the contestant's briefcase for a sum based on its expected value, given the information now at hand, but tweaked sometimes to make the game more interesting. The contestant can accept ("Deal") or opt to continue play ("No Deal").
- If the game continues, 5 more briefcases are opened in the second round, another offer is made, and accepted or refused. If the contestant continues to refuse the banker's offers, subsequent rounds open 4, 3, 2, 1, 1, 1, 1 briefcases until only two are left.
- The banker makes one last offer; the contestant accepts that offer or takes whatever money is in the initially chosen briefcase.
So the banker is always trying to buy out the player. If he fails, the player will end up with the amount in his briefcase.
The best way to understand the game is to play it here on the NBC website.
We did this, playing the only the first round. We chose number 13 for our briefcase. We were then asked to choose six briefcases. We chose briefcases 3,10,12,13,19, 25. Here is the result of this round:
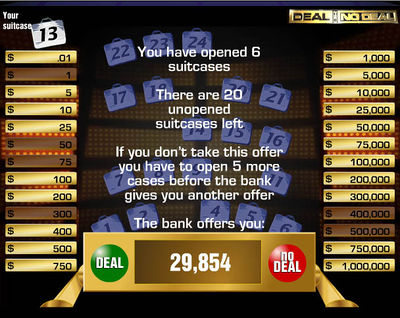
Our six choices eliminated the amounts 1, 50, 75, 300, 400,000, 500,000. The expected value of our briefcase was now the average of the amounts in the remaining briefcases. Making this calculation we found that this is $125,900 (rounded to the nearest dollar). The banker offered us $29,854 to quit. So we would have to have been pretty risk adverse to accept the banker's offer at this point.
In order to see if the banker's offers get better as we pick more briefcases, we played a second game in which we refused all the banker's offers. With this strategy we started with an expected winning given by the average of the initial amounts, which we found to be $131,478.
We then played all 9 rounds. In each round we recalculated the expected amount in our briefcase and recorded the banker's offer but refused it. Here are the results:
Round |
Expected Value |
Offer |
Percent of Expected Value |
1 |
130,857 |
30,565 |
23 |
2 |
142,475 |
37,361 |
26 |
3 |
125,186 |
54,507 |
44 |
4 |
153,319 |
57,554 |
38 |
5 |
170,967 |
67,160 |
39 |
6 |
205,160 |
108,950 |
53 |
7 |
256,425 |
176,781 |
69 |
8 |
341,800 |
317,700 |
93 |
9 |
512,500 |
367,500 |
72 |
We note that the banker's offer at each round was less than the expected amount in our briefcase. While it seemed clear at the beginning we would be right to refuse the offer, as we get nearer the end this is not so obvious. For example, after the 8th round the only amounts available were $400, $25,000, and $1,000,000. We were offered $317,700, a pretty nice amount of money. If we rejected it we would have a 2/3 chance of ending up with a relatively small amount and a 1/3 chance of getting the million dollars. We might well be sufficiently risk averse at this point to accept the offer. However, we didn't and the briefcase we chose had the $400. Now the only amounts left were $25,000 and $1,000,000. We were now offered $367,500. Again we might have thought twice about refusing this. But we did refuse it and we opened the last briefcase, which had the million dollars, So our briefcase had $25,000. If you watch the TV program you will find that most contestants refuse the banker's offers in the early rounds but accept it in one of the later rounds.
“Deal or No Deal” was introduced in Australia in 2003 and currently airs in 38 countries including the U.K. Those who feel that Excel is slow to fix its statistical errors will be amused by the Wikipedia account (See Predictable Sequence Controversy) of what happened to the UK “Deal no No Deal” game when the Excel random number generator was not properly seeded. Apparently the Excel error allowed one to estimate the contents of the remaining boxes--obviously very useful information.
This error was first reported by the U.K. Bother's Bar website. You can see the response of the “Deal or No Deal” producers of their home page (see Glenn Hugill writes). You can find an interesting discussion of the U.K. version of Deal or no Deal by the Brother's Bar here. In particular in their FAQ they give the following answer to question everyone asks: How does the Banker come up with the offers?
Banker's software throws up a lower and higher cash sum based on the values left on the board. The Banker then puts his own spin on the proceedings by picking a figure between the two boundaries. Factors such as the attitude of the player and who is having the better run of luck are all considerations. Producer Glenn Hugill says "I don't pretend to know how he operates but I believe there is a range which is considered to mathematically cover the financial risk. However if the Banker wishes to gamble or indeed play safe he can accordingly pitch his offer anywhere below or above it."
Bother's Bar also collected data which made it possible to compare the Box(Briefcase) value with the Banker's offer. You will find here the Box(briefcase) value, Peak bank offer, and Prize won for all programs from October 31 2005 to the present time.
For their Economics paper, Post and his co-authors obtained videos of 53 episodes from Australia and the Netherlands. From these they determined, for each contestant and each round, the situation the contestant faced in terms of the amounts of money in the remaining briefcases, the Banker's offer and their decision to accept or refuse the offer. The authors then analyze this data using a measure of relative risk aversion developed by Arrow and Pratt (RRA). They write:
In every game round, a unique RRA coefficient can be determined at which the contestant would be indifferent between accepting and rejecting the bank offer. If the contestant accepts the offer, his RRA must be higher than this value; if the offer is rejected, his RRA must be lower.
They also investigate how this RRA changes according to other variables such as estimated income and education.
The authors find that, for a wealth level of €25,000, an average over all these contestants gives an RRA 1.61. This estimate decreases when the wealth level decreases and increases when it increases. The degree of risk aversion differs strongly across the contestants, some exhibiting strong risk adverse behavior (RRA > 5) and others risk-seeking behavior (RRA < 0). They say:
The degree of risk aversion differs strongly across the contestants, some exhibiting strong risk averse behavior (RRA > 5) and others risk seeking behavior (RRA< 0). The differences can be explained in large part by the earlier outcomes experienced by the contestants in previous rounds of the game. Most notably, RRA generally decreases following losses. Contestants facing a large reduction in the expected prize during the game even exhibit risk-seeking behavior.
DISCUSSION
(1) Suppose that at each round the banker offered you the expected value of the amount in your briefcase and you were only interested in maximizing your expected winning. Would it matter when you stopped?
(2) Professor Post tells us:
The banker behaved in a predictable manner, with offers around 5-10% of expected value in round one and 100% in the later rounds. Also, for losers, the offers are relatively generous, sometimes exceeding 100%.
This leaves many questions about how the banker made his offers: Is there a real Banker or is it all done by an algorithm? The Bother's Bar’s comments suggest there is a real Banker for the U.K. program. Does the offer depend only on the expected amount in a player's briefcase? If you are teaching a probability or statistics course you could ask the students to look into one of these questions or to make their own hypothesis about how the offers are made.
(3) Suppose you knew how the offer is determined. How would this affect your strategy for playing the game?
(4) Suppose you are told that the banker knows what is in your briefcase. How would this affect the way you played the game?
(5) Using the Bother's Bar data, compare the sum of the prizes given out with the sum of the Box values when the prizes are awarded.
Submitted by Laurie Snell
Sexual prediction
Predicting the sex of fetus has been around for as long as children have been born. According to Roger Dobson of The Independent (March 7, 2006) there are many "theories from the wilder side."
- Coffee: If a man has coffee before sex, the Y-sperm is more active and likely to result in a boy.
- Dreams: Whatever sex of child a mother dreams of having, she will have the opposite.
- Age: As a mother gets older, her chance of conceiving a boy increases.
- Positions: Missionary position is best for a girl, says Italian folklore.
- Underwear: Men who wear loose underwear are more likely to produce boys.
And several others which we would also view as less than scientific. Now, however, modern methods of predictions with probabilities attached exist. Unfortunately, it is difficult to tell from the article whether it is P(X|Y) or P(Y|X). For example, "The Whelan method, named after Dr. Elizabeth Whelen," and depends on the timing of ovulation, "is claimed to be some 68 per cent effective for boys and 56 per cent for girls." Assuming that boys and girls are equally likely and "effective" means P(Test Boy|Boy) = .68, and P(Test Girl|Girl) = .56, translates via Bayesian inversion to a not very impressive P(Boy|Test Boy) = .61 and P(Girl|Test Girl) =.64.
"Another technique, the MicroSort method" which depends on DNA relating to the difference in size of the X and Y chromosomes, yields "success" rates of 88 per cent for girls and 73 per cent for boys. Assuming again that boys and girls are equally likely and "success" is synonymous with "effective," this translates via Bayesian inversion to a slightly more striking P(Boy|Test Boy) = .86 and P(Girl|Test Girl) =.77.
None of these methods can compare with that of the Acu-Gen Biolab which claims 99.9 per cent "accuracy," a term which sounds like "effective" or "success" but may not mean anything because some mothers who paid $25 for the kit and the $250 for the analysis wound up with a child of the other kind than what they were told. According to the Boston Globe (March 1, 2006), "Alleging that their pregnancies had been marred by fraud, 16 women have filed a class-action lawsuit against a Lowell laboratory that promises to determine the gender of an embryo by testing the mother's blood just five weeks after conception. In the suit filed in US District Court in Boston, the women charged that despite its claim of 99.9 percent accuracy, Acu-Gen Biolab of Lowell got the genders of their babies wrong, causing confusion and distress, and then refused to make good on its double-your-money-back guarantee."
Whether believer or buyer, always beware.
DISCUSSION
1. What are the ethical implications of an inexpensive, non-invasive sex prediction procedure which is available very early on in a pregnancy? What are the implications for the anti-abortion movement and for the pro-choice adherents?
2. Comment upon the linguistic dangers of using ordinary English, as in "effective," "success" and "accuracy" for well-defined statistical concepts.
3. Are you surprised that Acu-Gen Biolab "refused to make good on its double-your-money-back guarantee"? What excuses or reasons do you think the firm would give for claiming the fault lies with the woman and not with the technique?
Submitted by Paul Alper
Some children left behind
Joseph Stalin's famous quote regarding elections, "The people who count the votes decide everything" has an analogy to the bizarre world of the No Child Left Behind Act. Regardless of the merits of the act, it is in the interests of those who will be judged by this law to look good. As a consequence, it appears that there are several ways, other than outright cheating or the students actually testing higher, to make the numbers come out better.
In Minnesota, according to James Walsh of the Minneapolis Star Tribune (March 6, 2006), the trick is to have the low-scoring students take a different test. "To some, it may look like we're gaming the system" says Tim Vansickle, the Minnesota director of assessments. Minnesota does have many students whose native language is not English and Vansickle denies that the alternative test is not meant to help schools keep off "the list" of schools deemed failing.
Another technique according to Jay Mathews of the Washington Post (February 28, 2006), is to engage in strange arithmetic. He quotes Monte Dawson, the testing and assessment director for Alexandria, Virginia: "Remediation Recovery, which has been around since 2001, means that fourth grade students who failed the third grade test in 2004, got to retake the third grade test in 2005. Up until this year (2005), if they passed the third grade test, then they were included in the numerator only of the calculation to determine the third grade passing score. As illustration, if 4 out of 5 third grade students passed and 1 out of 5 fourth grade Remediation Recovery students passed, the passing percentage would be 100 percent."
Mathews, the Washington Post's education columnist, is puzzled by this explanation so he leaves it to the spokesman for the Virginia Department of Education, Charles Pyle. Mathews translates Pyle's remarks thusly: "in 2000, the state school board changed the counting procedure to encourage more schools to do what Maury [the school featured in the article] did -- give the students who failed some extra help and let them try again. Often the second-test passing rates of students who flunk a test initially are lower than their class's overall passing rate, since they are the class's weakest students. So if those second-test results were combined with the first test results in the usual way, it would likely lower the overall percentage and make the school look worse than otherwise. School districts in Virginia figured this out and resisted the urge to work with their lowest-performing students and test them again."
Therefore, to avoid this inevitable drop in performance should a school actually try to help its students rather than game the system, Mathews uses the following illustration: "To give schools an incentive to make that effort, the school board ordered an unorthodox change in the way the school percentage would be calculated after the retesting. If a school had 100 students, with 30 failing the test the first time and 10 of those passing the test the second time, they could add 10 to the 70 who passed the first time, divide those 80 passing students by 100, and get a nice boost from 70 to 80 percent in their passing rate. Done the conventional way, they would have had to add 30 to the denominator as they added 10 to the numerator, and gotten a passing rate of only 62 percent, lower than the 70 percent rate they had before."
Stalin was undoubtedly an evil person but his remark about defining and controlling the counting and evaluation procedure has many followers who probably don't realize the inspiration for their creativity. Perhaps the moral of the story for statisticians is the well-known dictum, "the data never speaks for itself." People have great ingenuity particularly when it comes to guarding their vested interests via number manipulation.
DISCUSSION
1. Is the purpose of the No Child Left Behind Act punishment of teachers and administrators or is the purpose to improve performance of the students?
2. Why is the Act controversial?
3. If the rules of the game allow the numerator to increase while not changing the denominator, what is there to complain about?
4. If you were a teacher or administrator would you be tempted to suggest to your poorer performing students to stay home on the day of the test?
Submitted by Paul Alper
Norton Starr sugested the next article.
The future divined by the crowd
The Future Divined by the Crowd
New York Times, March 11, 2006
Joe Nocere
Michael Mauboussin is a well known Wall Street strategist who also is an adjunct professor at Columbia Business School. Every year since 1993 he has asked his students to vote for the winners in 12 categories including major and relatively obscure contests. We read:
This year, the pick that got the most votes — the consensus pick, he calls it — turned out to be right in 9 of the 12 categories, including, amazingly enough, film editing and art direction. And yet, of the 47 students who participated, only one matched the accuracy of the consensus. None did better, and most did much worse; according to Mr. Mauboussin, the average number of correct answers per ballot this year was only 4.1. "It has never failed," he said. "The consensus invariably does much better than the average student."
The article also discusses other evidence that consensus predictions are often better than individual predictions such as the Iowa Electronic Market ((Chance News 13.06) , Holywood Stock Exchange (Chance News 12.02), and a new one HedgeStreet whose motto is: "It's your economy. Trade it."
DISCUSSION
(1) Choose one of the 12 categories and assume that there are 5 nominees for this category labeled 1,2,3,4,5. Assume that each of the 47 students rolls a five-sided die to determine their prediction. What is the probability that that their consensus vote is the Oscar winner for this category?
(2) Assume that there are 5 contestants for each of the 12 categories and the 47 students guess the winner in each category. What is the probability that their consensus vote is the Oscar winner in 9 or more of the 12 categories?
Science in 2020 – databases and statistics
Computing the future, The Economist, 25th March 2006
2020 – Future of Computing, Nature, 23rd February 2006.
Exceeding human limits, Stephen H. Muggleton, Department of Computing and the Centre for Integrative
Systems Biology at Imperial College London.
This week’s Nature has a series of news features and commentaries looking at how tomorrow’s computer technology will change the face of science.
For example, in Science in an exponential world, Alexander Szalay in the Department of Physics
and Astronomy, Johns Hopkins University and Jim Gray at Microsoft Research, San Francisco,
promote the use of database tools, such as ‘data cubes’,
to ‘see’ a new pattern or find a
data point that does not fit a hypothesis.
The authors claim that many scientists now ‘mine’ available databases, looking for new patterns and discoveries, without ever picking up a pipette. So the authors warn about a forthcoming data explosion:
computers also perform data analysis; Matlab, Mathematica and Excel are popular analysis tools. But none of these programs scale up to handle millions of data records — and they are primitive by most standards. As data volumes grow, it is increasingly arduous to extract knowledge. Scientists must labour to organize, sort and reduce the data, with each analysis step producing smaller data sets that eventually lead to the big picture.
Their two-page paper also discusses how scientists are increasingly analysing complex systems that require data to be combined from several groups and even several disciplines.
Data is shared across departments and time zones, and important discoveries are made by scientists and teams who combine different skill sets — not just biologists, physicists and chemists, but also computer scientists, statisticians and data-visualization experts.
This leads the authors to conclude that today’s graduate students need formal training in areas beyond their central discipline, such as data management, computational concepts and statistical techniques.
On the topic of using databases to handle the data deluge, they say:
the systematic use of databases has become an integral part of the scientific process. Databases provide tools to organize large data sets, find objects that match certain criteria, compute statistics about the data, and analyse them to find patterns. Many experiments today load their data into databases before attempting to analyse them. But there are few tools to properly visualize data across multiple scales and data sets. If we can no longer examine all the data on a single piece of paper, how can we ‘see’ a new pattern or find a data point that does not fit a hypothesis?
Another issue that is touched on is the reproducibility of any analysis. With experiments involving more people and data, documenting the data, software versions, models, parameter settings etc is now becoming a task best left to computers to record. But for the analysis to be repeatable in twenty years’ time the tools as well as the data will have to be archieved.
In another Nature commentary, Stephen Muggleton, the head of computational bio-informatics at Imperial College, London, claims that computers will soon play a role in formulating scientific hypotheses and designing and running experiments to test them. The data deluge is such that human beings can no longer be expected to spot patterns in the data. So the future of science involves the expansion of automation in all its aspects including data collection, hypothesis formation and experimentation.
For example, machine-learning techniques from computer science (including neural nets and genetic algorithms) are being used to automate the generation of scientific hypotheses from data. Some of the more advanced forms of machine learning enable new hypotheses, in the form of logical rules and principles, to be extracted relative to predefined background knowledge. One such development that Muggleton mentions is the emergence within computer science of new formalisms that integrate two major branches of mathematics: mathematical logic and probability calculus.
Mathematical logic provides a formal foundation for logic programming languages; probability calculus provides the basic axioms of probability for statistical models, such as bayesian networks. The resulting ‘probabilistic logic’ is a formal language that supports statements of sound inference, such as ‘The probability of A being true if B is true is 0.7’. Although it is early days, such research holds the promise of sound integration of scientific models from the statistical and computer-science communities.
Muggleton also mentions the growing trend of automatically feeding the results an experiment back into the model to improve the final results.
laboratory robots conducted experiments on yeast using a process known as ‘active learning’. The aim was to determine the function of several gene knockouts by varying the quantities of nutrient provided to the yeast. The robot used a form of inductive logic programming to select experiments that would discriminate between contending hypotheses. Feedback on each experiment was provided by data reporting yeast survival or death. The robot strategy that worked best (lowest cost for a given accuracy of prediction) not only outperformed two other automated strategies, based on cost and random-experiment selection, but also outperformed humans given the same task.
Questions
- How realistic is it to expect to “examine all the data on a single piece of paper”?
- The authors call for better visualisation tools but don’t mention any examples, either good or bad. Can you suggest reasons why they might have chosen to side-step this issue.
Further reading
- The full suite of Nature articles are available on line
- Visit News@nature.com's newsblog to read and post comments on the future of computing.
- Microsoft 2020 Science website
Submitted by John Gavin.